GenAI in Legal: Progress, Promise, Pain and Peril
In Brief
Our Chief Strategy Officer, Casey Flaherty, previewed our current thinking back in January. Casey was privileged to join Microsoft’s Lydia Petrakis and Ford’s Darth Vaughn & Whitney Stefko to kick off SKILLS 2024. Lydia, Darth, and Whitney had previously anchored invite-only roundtables LexFusion had convened to create a safe space for real talk, about GenAI. These closed-door sessions brought together doers of consequence who had moved beyond hype to actual GenAI deployment for legal use cases—to the point they could share practical perspectives and valuable lessons learned.
Casey opened the SKILLS session with a composite report from the frontlines:
At Length
The foregoing is a synopsis of LexFusion’s present perspective on the progress, promise, pain and perils of GenAI in the delivery of legal services. For those interested in a deeper dive, the long-form version follows.
Some history
In January 2023, LexFusion publicly predicted GenAI was poised to dominate the conversation [2]. January 2023 seems like a lifetime ago. It is easy to forget how many confidently dismissed ChatGPT as just another ephemeral novelty. In early March 2023, we penned a primer presciently entitled PSA: ChatGPT is the trailer, not the movie [3]. In late March, after GPT-4 had finally arrived, we revealed we’d been trading on insider information. In And Now, Our Feature Presentation: GPT-4 is Here, we shared that we had early access to GPT-4 and front-row seats to law departments/firms deploying GenAI for legal use cases—before ChatGPT had even dropped [4].
“If ignorance is bliss, knowledge is pain”
But if ignorance is bliss, knowledge is pain. We knew the discourse would be bad. But we did not appreciate it would be so excruciatingly repetitive. We should have. After all, we accurately assessed that expectations would maintain a permanent lead on execution. We fully recognized this expectation gap would create ample space for silliness. In March 2023, before GPT-4 was released, in ChatGPT is the trailer, not the movie, LexFusion warned:
We are in for a torrential downpour of hype
The mere presence of an LLM does not make an application effective and can make it dangerous
There will be a flood of garbage products claiming to deliver AI magic. This is a near certainty. Regardless of how useful well-crafted applications powered by LLMs may prove, there will be many applications that are far from well-crafted and are merely attempting to ride the hype train.
Even good tools will be misapplied because of failures to do the prerequisite process mapping, stakeholder engagement, future-state planning, and requirements gathering, not to mention process re-engineering, implementation, integrations, knowledge management, change management, and training.
LLMs will change much. But LLMs will not change everything, and the danger of automating dysfunction remains high.
While we predicted the pain, LexFusion persists in our position that GenAI cannot be dismissed as mere hype. In theory, it could be pure hype. Hype can exist in a vacuum—i.e., without any underlying substance. That is, we can experience hype with no underpinning inflection.
But the inverse is not true. We never enjoy genuine inflection points sans hype. No matter how legitimate the leap forward, there will be all manner of wild-eye predictions that seem silly, or pompously premature, in retrospect. This dynamic is, for lack of a better word, annoying. But righteous frustration does not obviate the import of the core advancement.
LexFusion remains convinced that GenAI represents the next in a series of compounding technological supercylces.
Our regular refrain: “This is happening. It will be done BY you or it will be done TO you.” But we also consistently concede that the hype tsunami is an unavoidable source of unnecessary pain. As Casey correctly whinged in March 2023, “I have never been more pre-annoyed.”
.jpg)
Closed-door GenAI sessions
We knew the discourse would be banal. GenAI would be new, and different, and confusing, and scary. But we did not accurately anticipate the rut of repetition.
Even now, conversations seem trapped at the 101 level. Low-information participants occupy a spectrum but seem to primarily fall into three distinct categories:
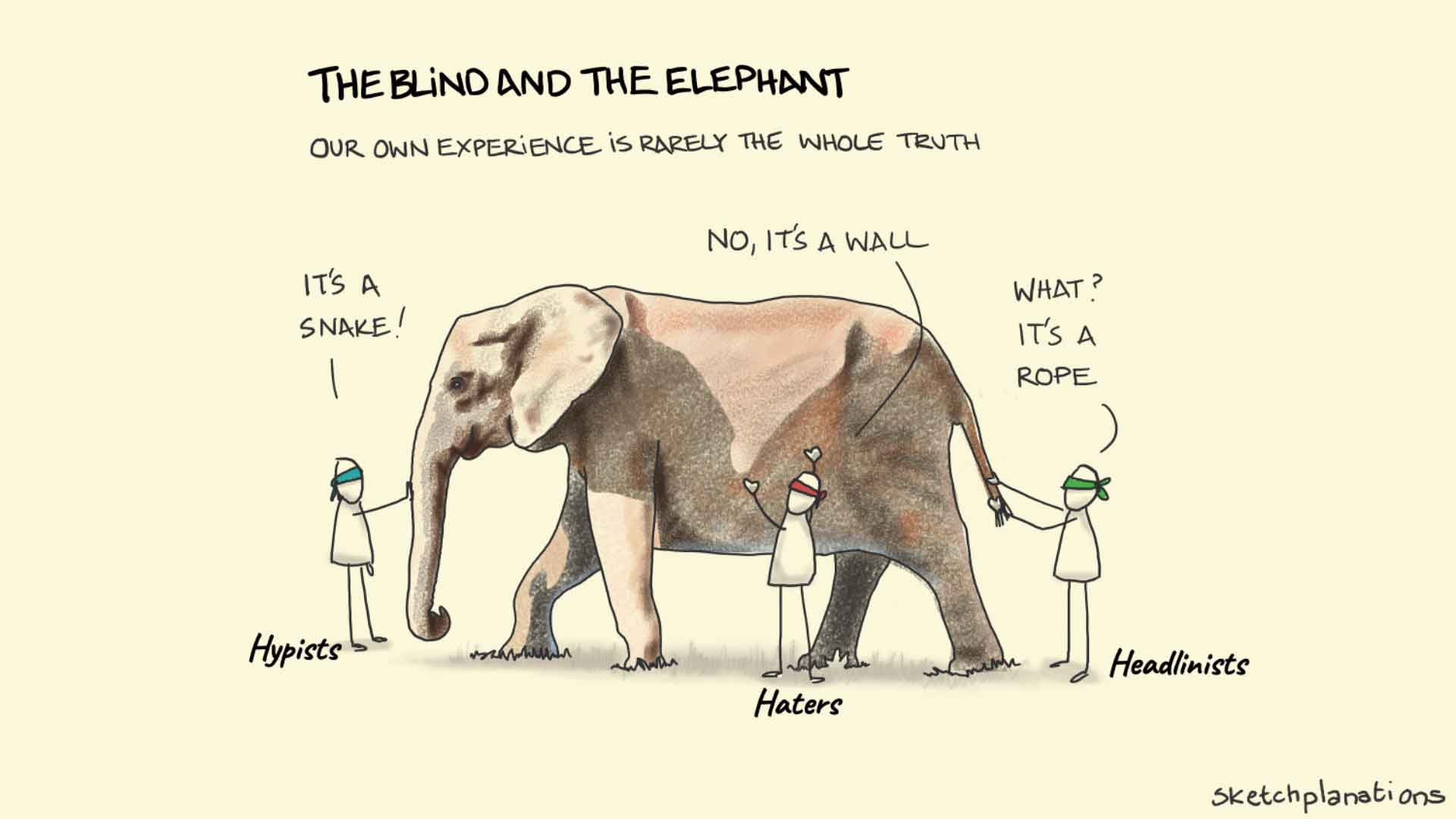
Annoyingly, they are not wrong. Any of them. There is much to be excited about (hypists). But there is also an attendant explosion of nonsense (haters). Both the opportunities and the nonsense represent real risks with respect to accuracy, privacy, cost, data security, explainability, intellectual property, etc. (headlinists).
Bringing low-information participants up to speed consumes so much bandwidth that the perpetually proliferating panels and webinars on GenAI rarely rise above the 101-level—vaguely exploring opportunities, overstatements, and obstacles. The discourse therefore suffers from a decided lack of specificity and practicality.

The criteria for speaking: to present at our initial sessions, an organization had to be (i) far enough along in its deployment of GenAI on legal use cases to have real lessons learned and (ii) willing to share their real-world experiences, positive and negative, to a degree specificity sufficient for their lessons learned to be useful to their sophisticated peers.
Happy talk and showerthoughts were expressly verboten. As were 101-level explainers. While presenters were welcome to reference legitimate issues like hallucinations, IP, and privacy, they were limited to doing so in the context of decisions they had actually made—e.g., we achieved acceptable accuracy by doing X…we did not extend the use case to Y due to [specific] privacy challenges we have yet to resolve.
LexFusion was so strict on quality control that we removed multiple prominent law departments and law firms from the agenda when they could not secure internal clearances to share at the required level of detail. Indeed, beyond welcome messages and housekeeping, LexFusion did not present at our own 2023 events because we did not meet the criteria—while we certainly use GenAI in our business, including this article, we do not yet have our own legal use cases (thankfully).

Spilling secrets
These closed-door events afford LexFusion the great privilege of targeted interactions with leading law departments and law firms. With such special access to industry pace setters, we are frequently asked to share the inside scoop about the amazing use cases the most mature organizations have uncovered.
Truth be told, the initial use cases are boring. As they should be. Everyone started with the obvious. Because of course they did.
Indeed, worse than boring, the truth is depressing. Turns out, GenAI is not magic. GenAI represents a genuine advancement. Ignoring GenAI would be foolish. Failure to start down the GenAI path is a terrible plan. But GenAI is a path, not a teleportation device. There is a staggering amount of work to be done to realize GenAI’s potential, especially in an enterprise environment.
Echoing the prevalent patter that long preceded the GenAI hype, the real people doing the real work emphasize the importance of prioritization, evaluation, planning, scoping, purposeful tradeoffs, knowledge management, change management, leadership buy-in, stakeholder engagement, data hygiene, training, maturation of the application layer, UI/UX…that is, the hard parts; the persistent, non-negotiable impediments to tech-centric improvement initiatives.
In short, the roundtable participants explained in a variety of ways that successful deployment of GenAI is dependent on successful projects. Worthwhile projects. Essential projects. But projects just the same. Projects, however, are problematic. Only 0.5% of projects deliver on time, on budget, and with the expected benefits [7].
We have no reason to expect GenAI projects will fare better.
Nitwits navigating a jagged frontier
In January 2024, Stanford released a pre-print study under the headline Hallucinating Law: Legal Mistakes with Large Language Models are Pervasive [8]. In short, the study found that no one should rely on raw large language models for legal work product.
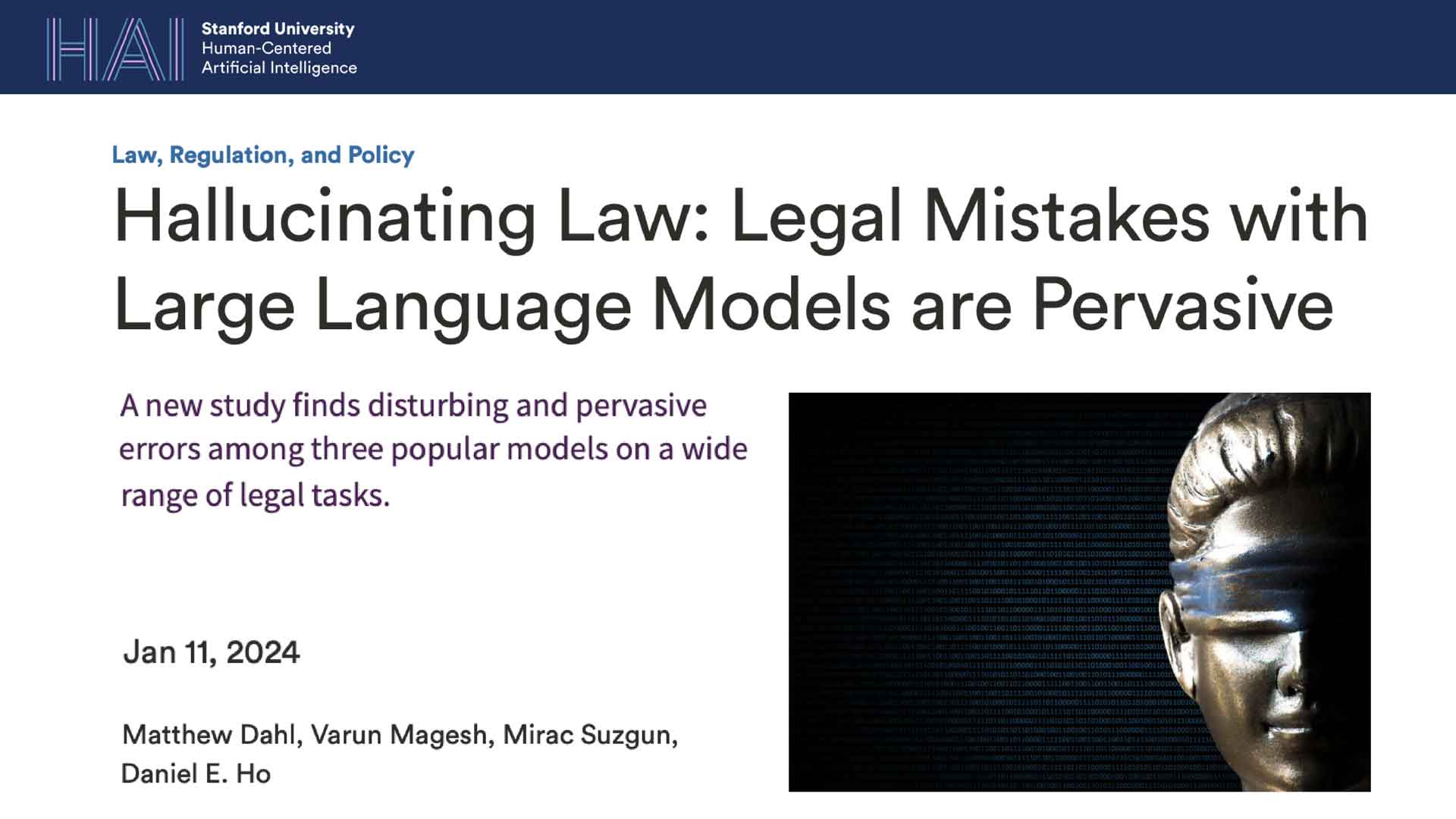
In a just, sane, rational world, we should be permitted to respond to this ‘revelation’ with abject sarcasm, “Thank you, Captain Obvious!”
Sure, in March 2023, Steven Schwartz, a small-time lawyer in New York was too cheap to spend the $95 to upgrade his free (through the state bar) Fastcase subscription to include federal content and, instead, submitted a brief in which he relied on fake precedents he had prompted ChatGPT to fabricate [9].
But that edge case already happened. It was widely publicized and criticized. Even in those bygone days, the way Schwartz kept doubling down—ultimately manufacturing the fictional opinions when opposing counsel and the court pointed out his citations did not exist—seemed to indicate nearly incomprehensible idiocy. Morons make marvelous martyrs because their extreme behavior often offers excellent exemplars of what not to do.
But, as it turns out, we do not live in a rational world, and information is not nearly as evenly distributed as foolishness. Nine months after his neighbor Schwartz’s ignominy and, less than two weeks before the release of the Stanford study, a notorious New York attorney-turned-client, Michael Cohen, passed on Bard’s counterfeit citations to his two defense attorneys, neither of whom bothered to check their veracity despite Cohen’s poor track record of reliability [10]. And the hits keep coming from lawyer after lawyer.
These are stories of incompetent lawyers, not incomprehensible tech. As Ford’s Whitney Stekfo astutely observed in our SKILLS presentation, the legal profession did not blame Zoom for the “I am not a cat” lawyer. Nor should we blame generalist tools like ChatGPT and Bard when they are abused by imbeciles.
Except, while the language above implies intellectual inadequacies, the immediate issues are more about ignorance, intuition, and indolence (i.e., laziness).
The ignorance is endemic. Only 23% of adults have used GenAI [11]. These low usage rates include lawyers [12]. Yet, at the individual and organizational level, GenAI demands a fair amount of learning by doing [13]. Unfortunately, our culture of experimentation and continuous learning in legal is badly broken [14].
One reason learning by doing is imperative is that GenAI is far from intuitive. Operating in ignorance, it is not shocking that Schwartz, Cohen, et al. would treat AI chatbots as supercharged search engines able to locate and synthesize pertinent information—indeed, retrieval augmented generation is a common use case for GenAI, especially in legal.
But the counterintuitive aspects of GenAI are even more subtle.
Ethan Mollick, author of the must-follow One Useful Thing substack and the must-read book Co-Intelligence, is a professor at Wharton, where his focus is innovation, entrepreneurship, and the effects of AI on work & education. In September 2023, he was part of a Harvard team that published the most revealing study to date on the use of AI in knowledge work. Entitled Navigating the Jagged Technological Frontier: Field Experimental Evidence of the Effects of AI on Knowledge Worker Productivity and Quality, the study measured the performance of 758 Boston Consulting Group consultants across 18 complex, knowledge-intensive tasks [15].
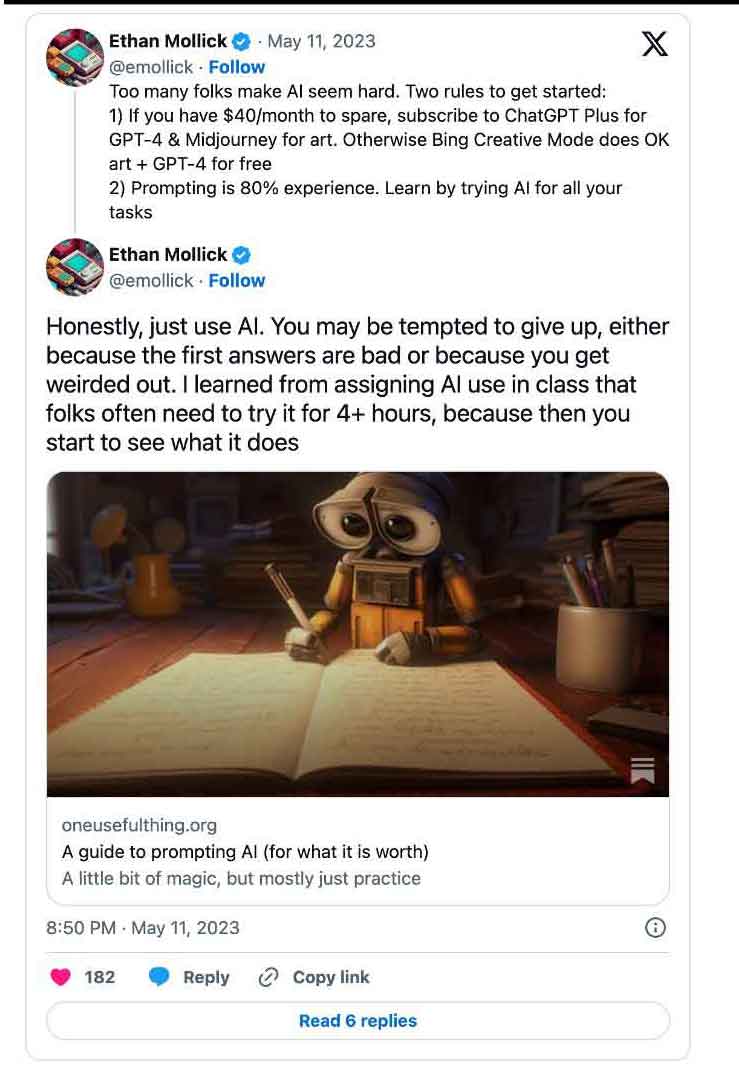
The findings command attention:
For each one of a set of 18 realistic consulting tasks within the frontier of AI capabilities, consultants using AI were significantly more productive (they completed 12.2% more tasks on average, and completed task 25.1% more quickly), and produced significantly higher quality results (more than 40% higher quality compared to a control group). Consultants across the skills distribution benefited significantly from having AI augmentation, with those below the average performance threshold increasing by 43% and those above increasing by 17% compared to their own scores.
But the underlined qualifier—“within the frontier of AI capabilities”—is critical to correctly capture the crux of the research. The study further found:
For a task selected to be outside the frontier, however, consultants using AI were 19 percentage points less likely to produce correct solutions compared to those without AI.
Mollick artfully articulates the concept of the “frontier” in an accompanying post [16]:
AI is weird. No one actually knows the full range of capabilities of the most advanced Large Language Models, like GPT-4. No one really knows the best ways to use them, or the conditions under which they fail. There is no instruction manual. On some tasks AI is immensely powerful, and on others it fails completely or subtly. And, unless you use AI a lot, you won’t know which is which.
The result is what we call the “Jagged Frontier” of AI. Imagine a fortress wall, with some towers and battlements jutting out into the countryside, while others fold back towards the center of the castle. That wall is the capability of AI, and the further from the center, the harder the task. Everything inside the wall can be done by the AI, everything outside is hard for the AI to do. The problem is that the wall is invisible.
“AI is weird…The wall is invisible.” AI helps quite a bit. Except where it doesn’t. In which case, AI makes us worse. Oh, and the dividing line between helps/hurts is jagged, invisible, and non-intuitive.
Plus, we might be too lazy to prudently navigate the jagged frontier—i.e., ignorance + non-intuitiveness + indolence. As Mollick writes:
Fabrizio Dell’Acqua shows why relying too much on AI can backfire. In an experiment, he found that recruiters who used high-quality AI became lazy, careless, and less skilled in their own judgment. They missed out on some brilliant applicants and made worse decisions than recruiters who used low-quality AI or no AI at all. When the AI is very good, humans have no reason to work hard and pay attention. They let the AI take over, instead of using it as a tool. He called this “falling asleep at the wheel”, and it can hurt human learning, skill development, and productivity.
In our experiment, we also found that the consultants fell asleep at the wheel – those using AI actually had less accurate answers than those who were not allowed to use AI (but they still did a better job writing up the results than consultants who did not use AI). The authoritativeness of AI can be deceptive if you don’t know where the frontier lies.
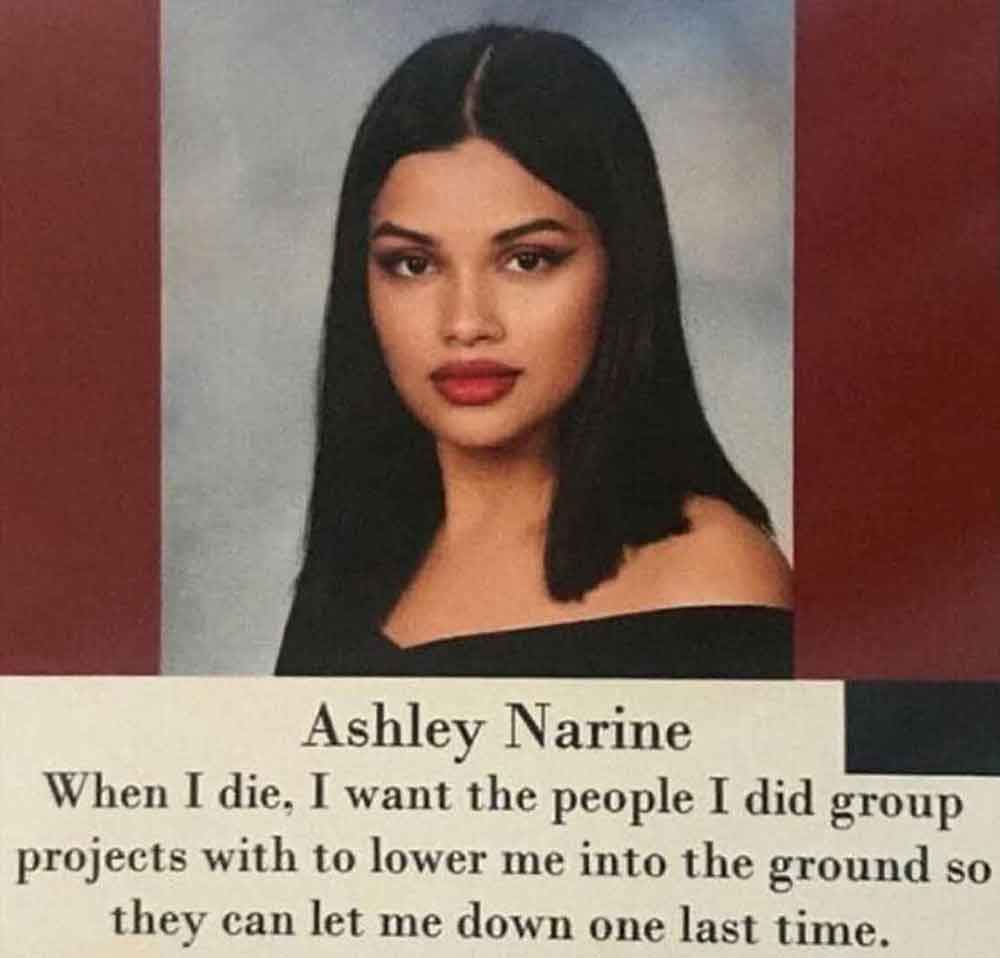
How we team with AI is another aspect of properly navigating that invisible, non-intuitive, jagged frontier. As Mollick explains, the research suggests two distinct modes:
But a lot of consultants did get both inside and outside the frontier tasks right, gaining the benefits of AI without the disadvantages. The key seemed to be following one of two approaches: becoming a Centaur or becoming a Cyborg….
Centaur work has a clear line between person and machine, like the clear line between the human torso and horse body of the mythical centaur. Centaurs have a strategic division of labor, switching between AI and human tasks, allocating responsibilities based on the strengths and capabilities of each entity….
On the other hand, Cyborgs blend machine and person, integrating the two deeply. Cyborgs don't just delegate tasks; they intertwine their efforts with AI, moving back and forth over the jagged frontier.
Humans
or modern humans (Homo sapiens), are the most common and widespread species of primate. A great ape characterized by their hairlessness, bipedalism, and high intelligence, humans have a large brain and resulting cognitive skills that enable them to thrive in varied environments and develop complex societies and civilizations.
(Wikipedia)
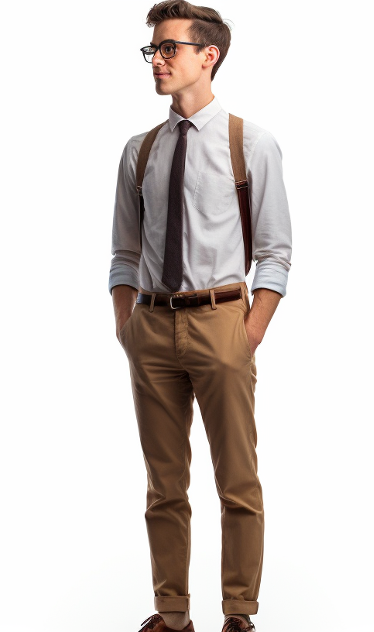
Centaur
work has a clear line between person and machine, like the clear line between the human torso and horse body of the mythical centaur. Centaurs have a strategic division of labor, switching between AI and human tasks, allocating responsibilities based on the strengths and capabilities of each entity.
(Ethan Mollick)
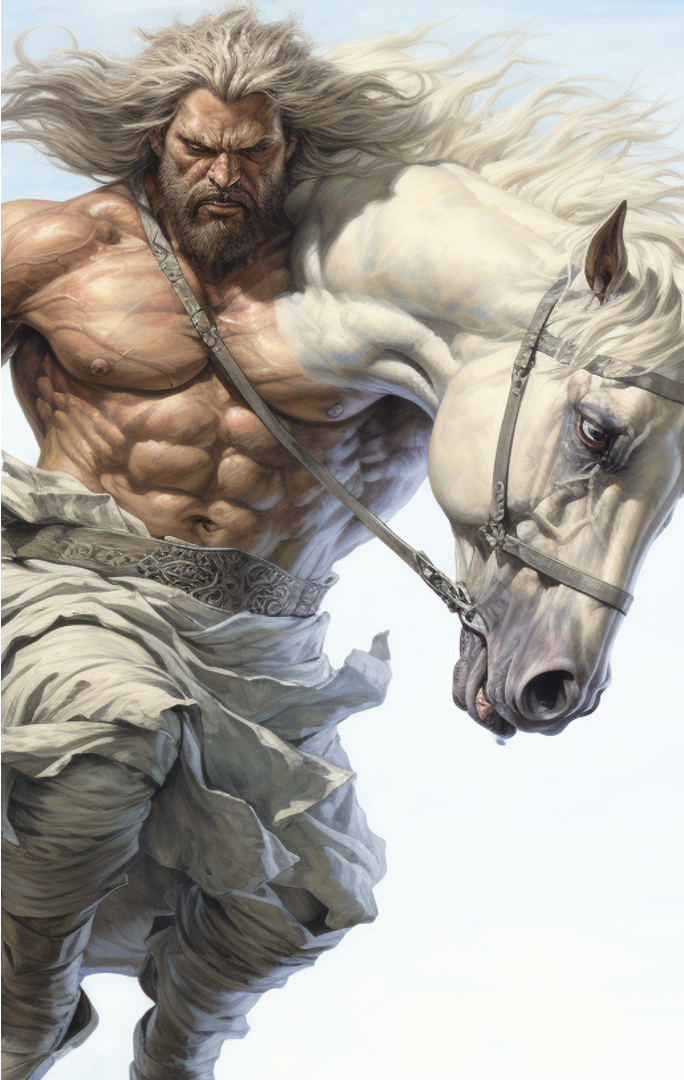
Cyborgs
blend machine and person, integrating the two deeply. Cyborgs don't just delegate tasks; they intertwine their efforts with AI, moving back and forth over the jagged frontier. Bits of tasks get handed to the AI, such as initiating a sentence for the AI to complete, so that Cyborgs find themselves working in tandem with the AI.
(Ethan Mollick)
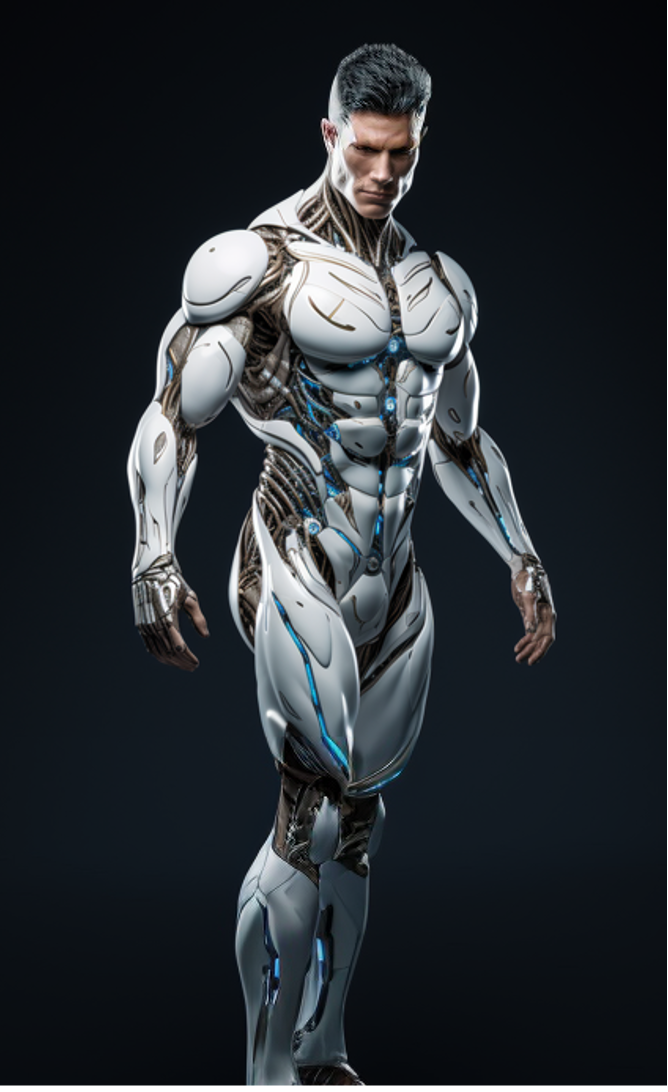
Everything should be made as simple as possible, but not simpler. Unfortunately, AI is not nearly as simple as we might prefer. But as confounding as this reality may be, AI cannot be ignored. As Mollick concludes:
regardless of the philosophic and technical debates over the nature and future of AI, it is already a powerful disrupter to how we actually work. And this is not a hyped new technology that will change the world in five years, or that requires a lot of investment and the resources of huge companies - it is here, NOW… the question is no longer about whether AI is going to reshape work, but what we want that to mean. We get to make choices about how we want to use AI help to make work more productive, interesting, and meaningful. But we have to make those choices soon, so that we can begin to actively use AI in ethical and valuable ways.
But we don’t want to make choices. What if we’re wrong?
We don’t want to experiment and learn. Who has time for that?
We want AI magic, not some invisible, jagged frontier. We want AI to do our work for, not with, us—Schwarz’s and Cohen’s original sins were more sloth than stupidity.
The best joke at our closed-door sessions came from a prominent head of innovation at a major law firm (paraphrasing), “The lawyers fear the AI is good enough to replace them and then, when we show them the tools, are deeply disappointed the AI is not nearly good enough to replace them.” That is, there is an implicit expectation that for the AI to really be AI, it must be superhuman—better than all humans at all tasks. The disappointing truth is that, today, it is better than some humans at some tasks—co-intelligence rather than super intelligence.

Put another way, tell a lawyer that an AI-enabled tool will get them 80% down the road towards useful work product, and these professional issue spotters will explain, in excruciating detail, that 20% is missing. Meanwhile, in many instances, the desired 100% substitution of machine for human is impossible, and getting anywhere close requires a considerable amount of work.
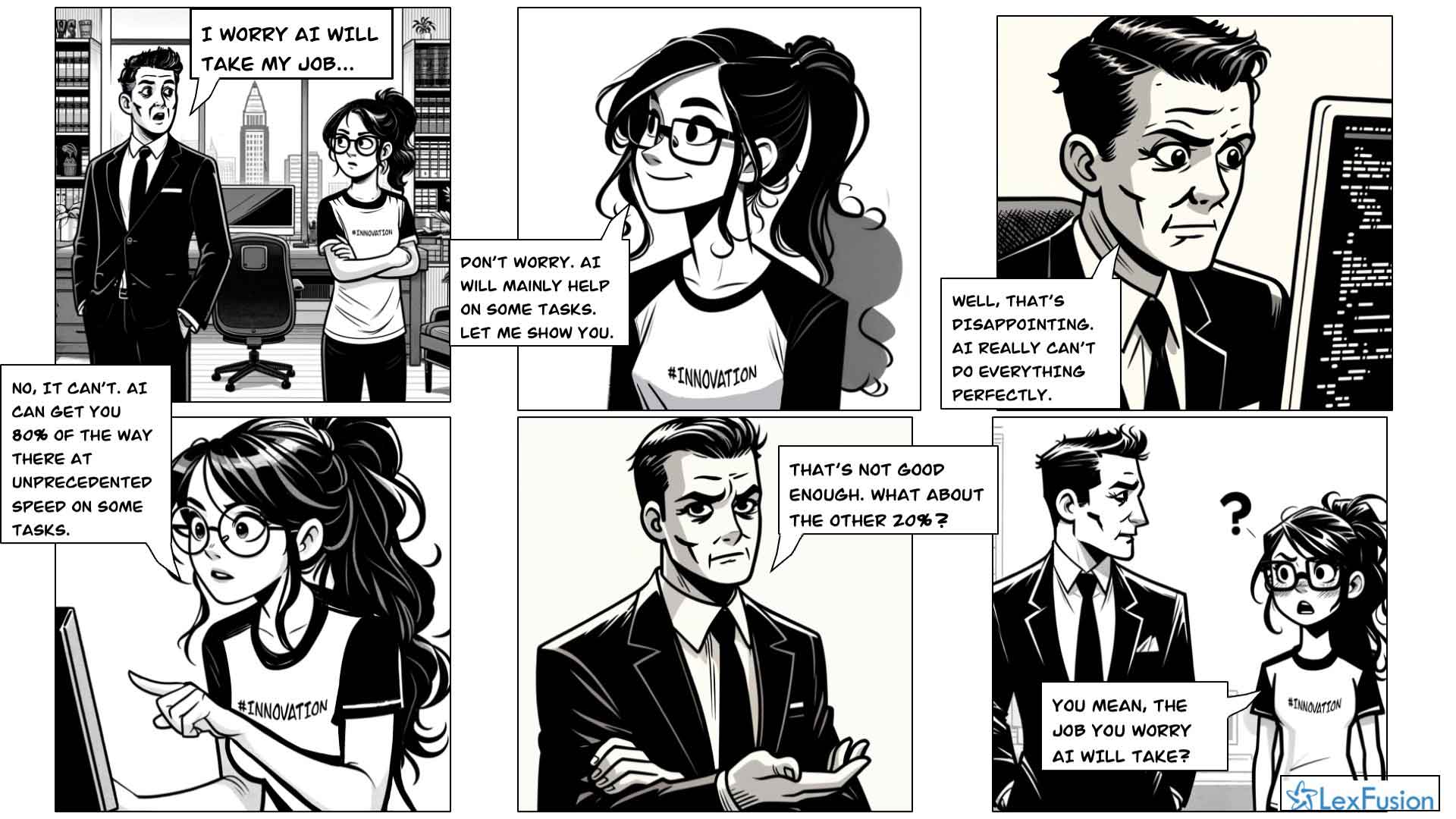
The road to product is long
GenAI is different, in part, because the underlying models are probabilistic, rather than deterministic [18]. This makes the models fantastically flexible, especially in processing the elaborate nuances of human language. Being probabilistic also makes the models prone to hallucination, among other shortcomings [19]. Unchecked, the models will always produce (statistically most likely) answers, even if the answers are (factually) wrong. We usually don’t need mere answers; we need truth.
Part of dealing with hallucinations is changing the way we work. Complementing the Jagged Frontier paper is research from Github that gives us a glimpse into the near future.
Github released their AI pair programmer Github Copilot in October 2021—more than a full year before the ChatGPT frenzy. By June 2023, 92% of U.S.-based developers were using AI coding tools and reporting material, measurable productivity improvements [20]. But AI does not merely increase human developer speed and quality, it changes the way developers work. Specifically, AI pair programmers “introduce new tasks into a developer’s workflow such as writing AI prompts and verifying AI suggestions.” [21].
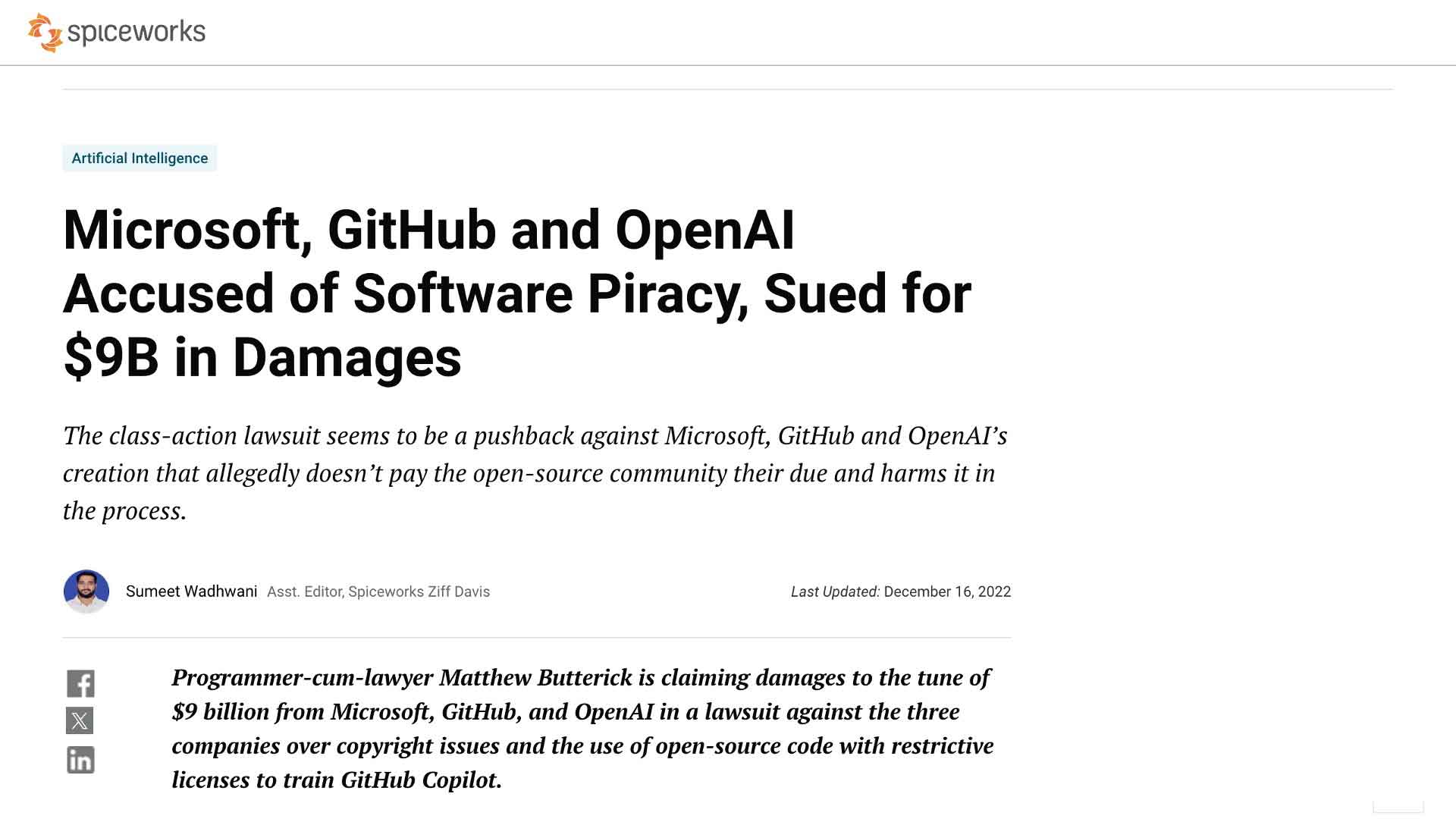
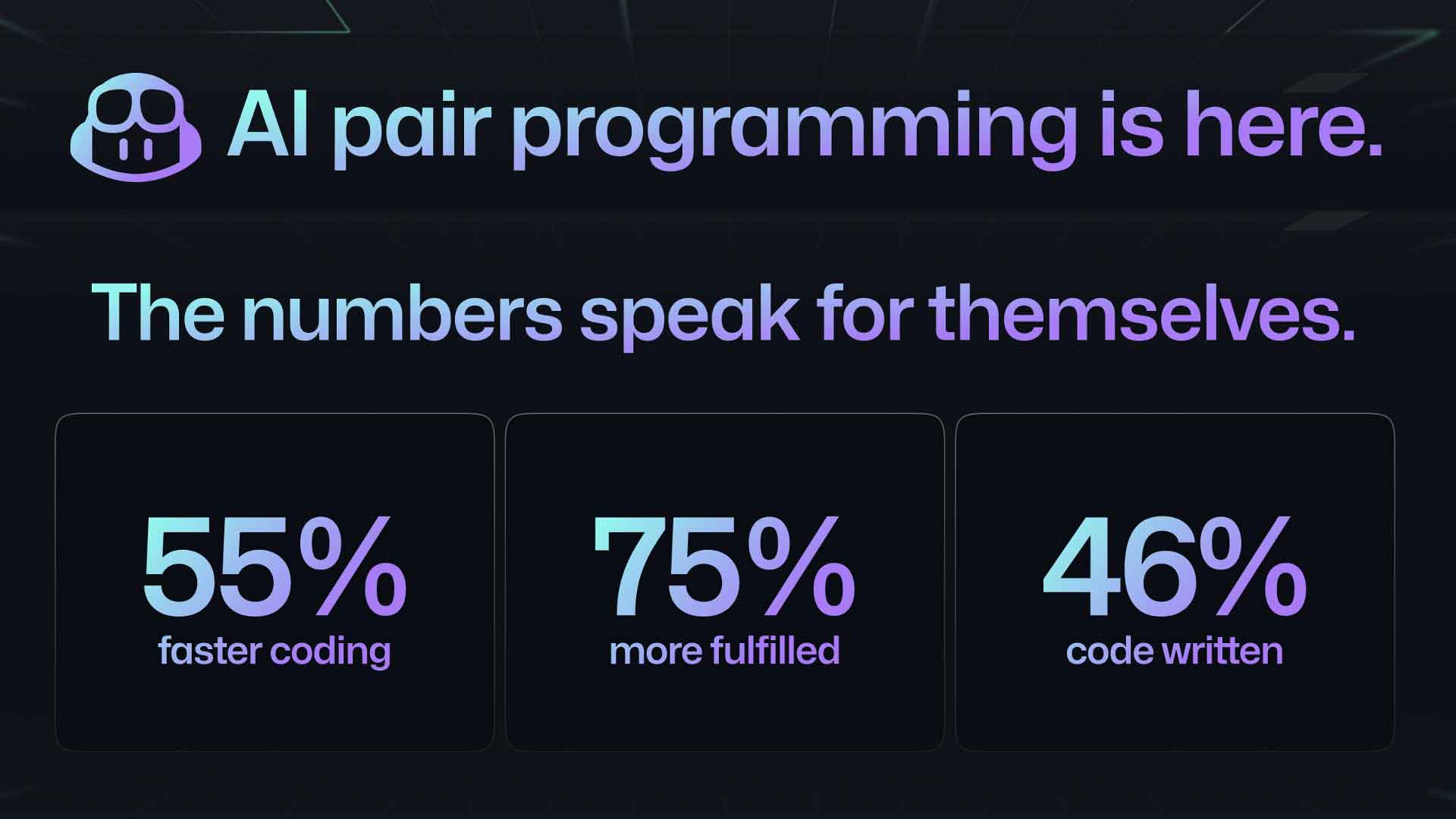
Github Copilot is more than a general large language model accessible through a thin chat layer. Github Copilot was based on Codex, a descendent of GPT-3 augmented by “billions of lines of source code from publicly available sources” [22]—that code being at the center of the now-narrowed lawsuits seeking $9+ billion from OpenAI, Github, and Microsoft (Github’s parent) [23]. Moreover, Github Copilot is an extension for Microsoft Visual Studio—i.e., it integrates directly into developers’ workflows. Github Copilot is carefully crafted to be fit for purpose and minimize (not eliminate) unnatural acts in the form of deviations from existing ways of working.
Bringing together a quality user experience with acceptable accuracy is much harder than it appears. Elegance comes from the hard engineering work of making the complex simple from the user perspective.
As a digestible example, LexFusion has been privileged to see elegance in action at Macro, which has brought a GenAI chat experience directly into a fully functional DOCX/PDF editor.
You may have read that Adobe has done the same with Acrobat, their ubiquitous PDF application. Indeed, both Macro and Adobe rely on Microsoft’s OpenAI Services to power their in-document GenAI chat [24]. The competing programs are applying the same GenAI model. To the same documents. With wildly different results.
Why? Same document. Same questions. Same model. It is not that Acrobat is bad. Rather, Acrobat is general purpose, whereas Macro is fit-to-purpose.
GenAI has many shortcomings beyond hallucinations. Context limits put a cap on, among other things, the length of documents that can be processed [25]. Yet larger context windows tend to degrade performance. Evidence suggests LLMs mirror the human habit of skimming and the human frailty of forgetting what we just read. Yes, really. Calling back to Mollick, AI is weird. The title of the most relevant paper is Lost in the Middle: How Language Models Use Long Contexts [26]. The conclusion:


Current language models do not robustly make use of information in long input contexts. In particular, we observe that performance is often highest when relevant information occurs at the beginning or end of the input context, and significantly degrades when models must access relevant information in the middle of long contexts, even for explicitly long-context models.
There are a variety of methods, like chunking, for breaking up documents to overcome these limitations [27]. Macro was well positioned to expertly employ such methods because their pre-existing, non-generative AI already deconstructs (well, graphs) documents in a logical fashion. That is, the syntactic parsing that enables Macro to turn cross-references into clickable hyperlinks also enables coherent chunking so documents can be processed by an LLM in a properly sequenced and cost-effective manner.
The foregoing is a simplified version of a seemingly simple GenAI use case. Yet, even in the simplified version, achieving elegance requires:
- A pure source of truth (here, the document itself)
- A clean, intuitive, integrated interface that enables the user to remain within a unified workflow
- Complementary AI (syntactic parsing) to enable logical chunking, maintaining performance while overcoming context limits and performance degradation
- Engineered prompts, running in the background, to mitigate hallucinations and ensure consistent accuracy
Macro founder Jacob Beckerman wrote his thesis on syntactic parsing and the graphing of complex documents [28]. He’s been working on productizing his research for years. That work has allowed Macro to become a prime example of a “thick wrapper” application [29].
As has been observed elsewhere, now that LLMs exist, an LLM is the easiest part of an LLM-enabled application [30]. Because the LLM part is relatively easy, we’ve had a Tribble-like explosion of “thin wrapper” applications where, in essence, the product is merely another access point, a simple overlay, to a commercially available LLM like GPT-4. But, even with LLMs, it is not easy to produce Easy Buttons, and, as LexFusion predicted, there has been an avalanche of garbage products.
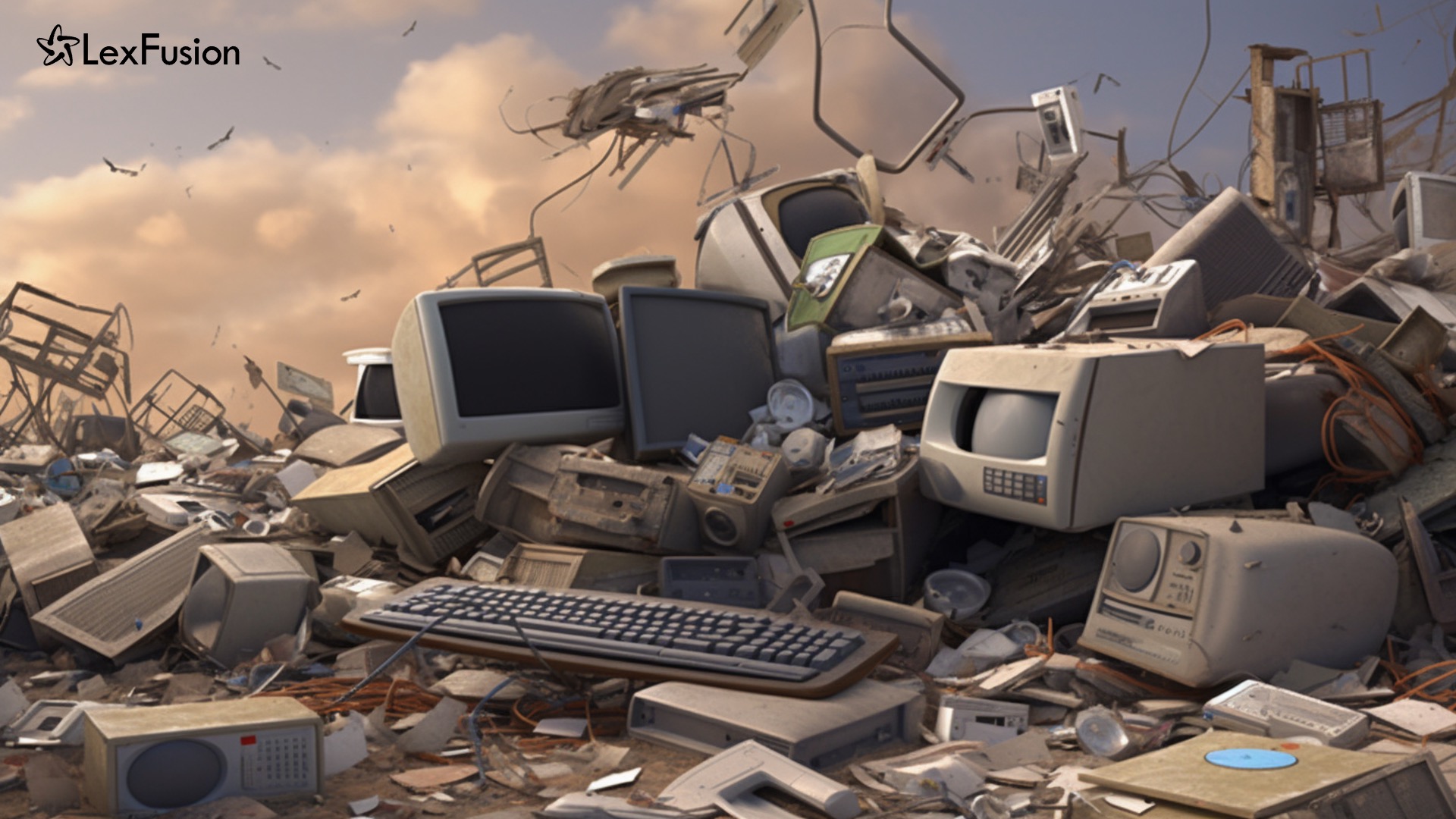
As Casey’s personal GPT explains it (unedited, except formatting; and, of course, being Casey’s GPT, it blew the word limit):
PROMPT: In under 500 words, explain the difference between thin wrapper and thicker wrapper applications, including why thick wrapper applications will often be more performant at specialized tasks in complex domains
Thin-Wrapper Applications
Thin-wrapper applications are essentially interfaces that provide access to a large language model (LLM) or other AI models with minimal additional functionality or customization. These applications often rely heavily on the raw capabilities of the underlying AI model without substantial augmentation. Examples include basic chat interfaces or simple query tools that feed prompts to a model like GPT-4 and display the output.
Advantages of thin-wrapper applications
1. Speed to Market: Because they require minimal development, thin wrappers can be deployed quickly.
2. Flexibility: They offer a general-purpose interface that can handle a wide range of tasks.
3. Cost-Effective: Lower development and maintenance costs due to their simplicity.
Disadvantages of thin-wrapper applications
1. Limited Customization: They often lack the specific features and optimizations needed for specialized tasks.
2. Performance Issues: Without additional layers of processing or domain-specific tuning, their performance can be inconsistent.
3. User Experience: They might not integrate seamlessly into existing workflows, leading to a disjointed user experience.
Thick-Wrapper Applications
Thick-wrapper applications, on the other hand, involve a more extensive integration of the AI model with additional tools, features, and customizations tailored to specific use cases. These applications are designed to optimize the performance of the AI model for particular tasks by addressing its inherent limitations and enhancing its capabilities with domain-specific knowledge and processing layers.
Advantages of thick-wrapper applications
1. Specialization: By incorporating domain-specific optimizations, thick wrappers can significantly enhance the accuracy and reliability of AI outputs for specialized tasks.
2. Performance: They integrate additional processing layers, such as prompt engineering, data preprocessing, and post-processing, to improve the model's performance.
3. Seamless Integration: Thick wrappers are designed to fit into existing workflows, offering a more intuitive and productive user experience.
4. Enhanced Capabilities: They often include complementary technologies like retrieval-augmented generation (RAG), which helps manage large datasets and improves the AI's ability to handle complex queries.
Disadvantages of thick-wrapper applications
1. Development Time and Cost: Creating a thick wrapper application requires significant investment in time and resources.
2. Complexity: They are more complex to maintain and update due to the additional layers of functionality.
Why Thick Wrappers Perform Better in Complex Domains
In complex domains, tasks often require more than just raw language processing capabilities. They need contextual understanding, integration with specific datasets, adherence to domain-specific standards, and the ability to handle nuanced queries. Thick-wrapper applications address these needs through:
1. Contextual Awareness: By incorporating domain-specific data and processing layers, thick wrappers can better understand and respond to context-specific queries.
2. Enhanced Accuracy: They use advanced techniques like prompt engineering and RAG to improve the accuracy of responses, reducing the likelihood of errors and hallucinations.
3. Workflow Integration: Thick wrappers are designed to integrate seamlessly with existing tools and processes, enhancing productivity and user satisfaction.
4. Robustness: They can handle the intricacies of specialized tasks by leveraging additional tools and optimizations, making them more reliable and effective.
In summary, while thin-wrapper applications offer a quick and flexible way to utilize AI models, thick-wrapper applications provide the necessary depth, accuracy, and integration required for specialized tasks in complex domains. This makes thick wrappers the preferred choice for achieving high performance and reliability in such environments.
Thick wrappers take time. The road to robust LLM applications is therefore long. Macro’s ‘shortcut’ was years of work on syntactic parsing that turned out to be synergistic with LLMs. Still, the days of thin wrappers seem numbered as they are displaced by fit-to-purpose thick wrappers like Macro and direct LLM pathways like Microsoft Azure, Amazon Bedrock, and Google’s Vertex AI.
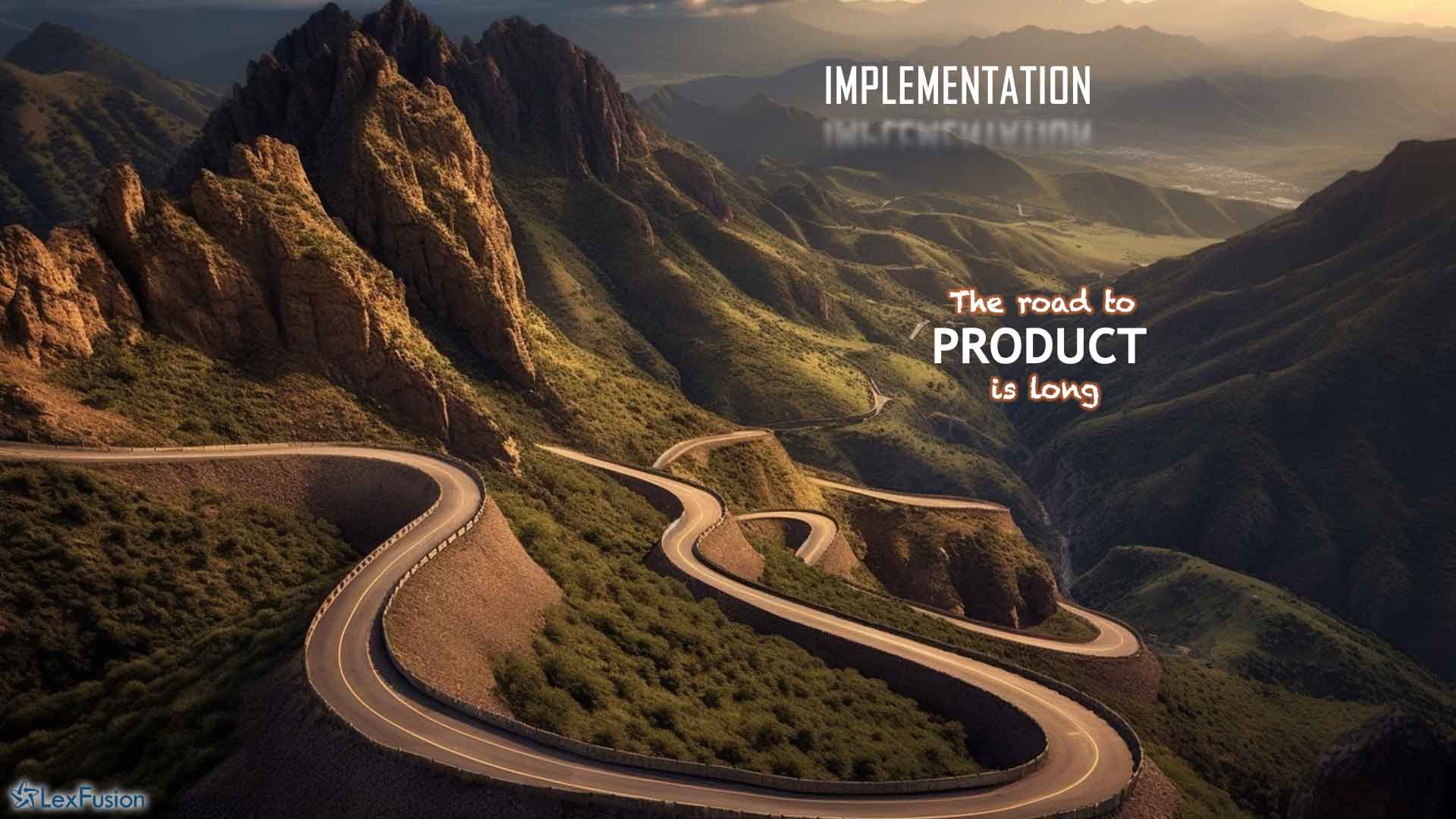
Enter the Dragon
To dive deeper into the requirements for a performant, thick-wrapper application, let us return to the innocent days of fall 2022 when LexFusion was working on an ambitious project to map the dealtech landscape. Casey’s presentation to the ABA M&A Technology Subcommittee detailed these efforts, which we abandoned immediately after our first sneak peek of GPT-4—we knew the game had changed.
As Casey explained at the time, tech had deeply disappointed lawyers for decades with respect to the holy grail that is an automated deal-points database—a comprehensive view of the critical provisions in transactional agreements that can provide insight into ‘what’s market’. Firms have long understood deal points as a competitive advantage in winning work, both showcasing experience and because of the high value for drafting and negotiation. But the labor- and time-intensive capture process limited firms’ capacity to manually build databases. Historically, only a few firms had the will and wherewithal to maintain a robust database. While important advances like Kira made material progress in clause abstraction and proved useful in a number of areas, especially due diligence, the dream of a deal-points database being accurately populated by a machine remained as elusive as it was desirable.
Deal points being so difficult for the machine to identify is an example of how AI has always been weird. Where deal specifics—dates, durations, dollar values, party names—are relatively easy for humans to recognize, writing comprehensive deterministic rules around complex concepts is a monumental challenge because of the subtle variations in where and how specifics are memorialized. Most deal points are nuanced provisions involving information compiled from multiple sections of text containing variations on analogous defined terms. Slight shifts in text can have big impact on ultimate meaning.

Probabilistic LLMs seemed a promising new angle on an old problem. The early returns, however, while positive, were not quite inspiring [31]. In their raw form, the most powerful LLMs proved somewhat accurate at extracting deal points. But ‘somewhat accurate’ is not nearly good enough. Extremely accurate is the threshold required to displace the labor-intensive surveys that few firms have ever had the discipline to fill out consistently despite the immense value an up-to-date deal-points database affords deal lawyers in yelling back and forth about ‘what’s market’.
That is, while deal-points extraction was an obvious use case, a thick wrapper was required to achieve an acceptable level of accuracy and cross the chasm of usefulness. Enter the Dragon.
Foundation Dragon is a new product from Haley Altman, Brett Balmer, and Litera. In its real-world pilots Dragon has achieved robust accuracy across hundreds of fields and multiple deal types—far exceeding not only any other tech but also any reasonable expectation of human performance (keep this point in mind: reasonable expectation of human performance).
Haley is a former M&A partner turned legaltech founder. Her co-conspirator, Brett Balmer is a technical wizard with deep experience in legal databases, having been the chief architect of Foundation. The importance of true subject matter expertise and the ability to translate latent knowledge into a performant product is hard to overstate [32].
As Haley permitted Casey to share with the SKILLS audience and then shared herself at the April 2024 LexFusion event hosted by Google in London, the Dragon team had to do the hard work of overcoming the inherent shortcomings of LLMs, including the aforementioned context limits, cross-references, and definitions.
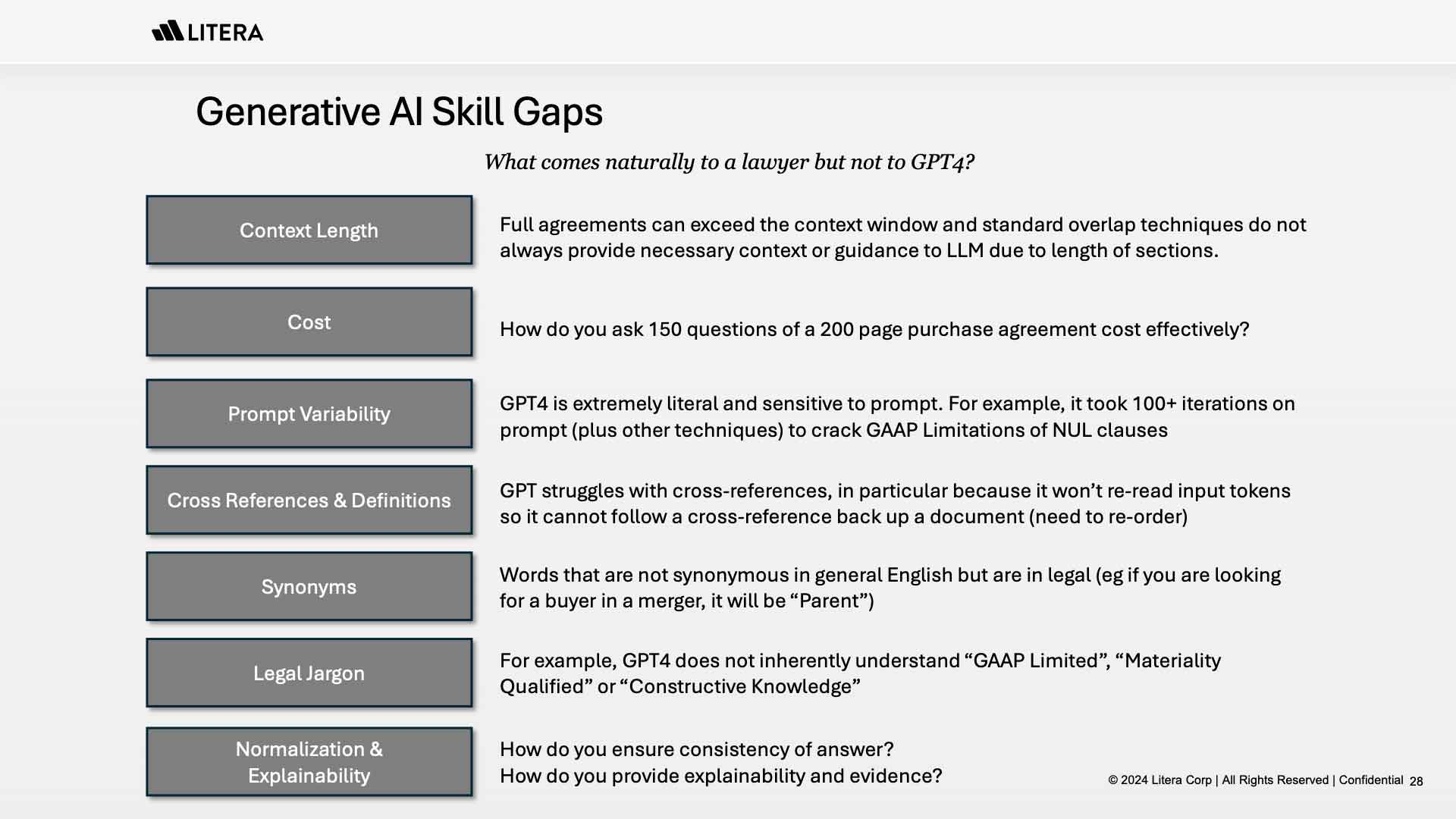
Haley and the Litera team turned pro at prompt engineering [33]. Even then, combining true experts in both the subject matter and prompting, required 100+ iterations of single prompts, combined with other Balmer-crafted techniques, just to crack some more tedious provisions, including Financial Reps with GAAP Limitations of NUL (no undisclosed liabilities) clauses.
While Haley would, understandably, not share Dragon’s final engineered prompt, nor the remainder of Balmer’s secret sauce, the subtle differences between a “not-so-good” prompt and a “better” prompt should reinforce how nuanced, and weird, working with GenAI can be.
In summary, consistent automated extraction of a single deal point required hours upon hours of work by a technologically proficient subject matter expert, supported by a technical wizard with a high level of domain knowledge. Those dozens of hours will result in many tens thousands of hours of time savings for Dragon users—the juice is worth the squeeze. But those material gains in efficacy are the product of work, not mere AI magic.

The ROI is substantial. But the work was essential. From a pure numbers perspective, the gap between Dragon and the raw, zero-shot performance of GPT-4 is material but not massive. From an “actually useful in legal practice” perspective, the gap is effectively infinite.
Yet, while an exponential improvement over the status quo ante, Dragon does not eliminate the expert in the loop. Dragon is still fundamentally premised on a survey process. The tech merely prepopulates the survey for the assigned expert (often a senior associate who orchestrated the deal) to verify deal points, including catching errors—perfection being an impossible standard for both unaided lawyers and unmonitored tech. Without Dragon, the surveys demand anywhere from 4 to 15 hours (depending on the deal type). With Dragon, that shrinks from 15 to 30 minutes. Magnificent. But not quite magic.
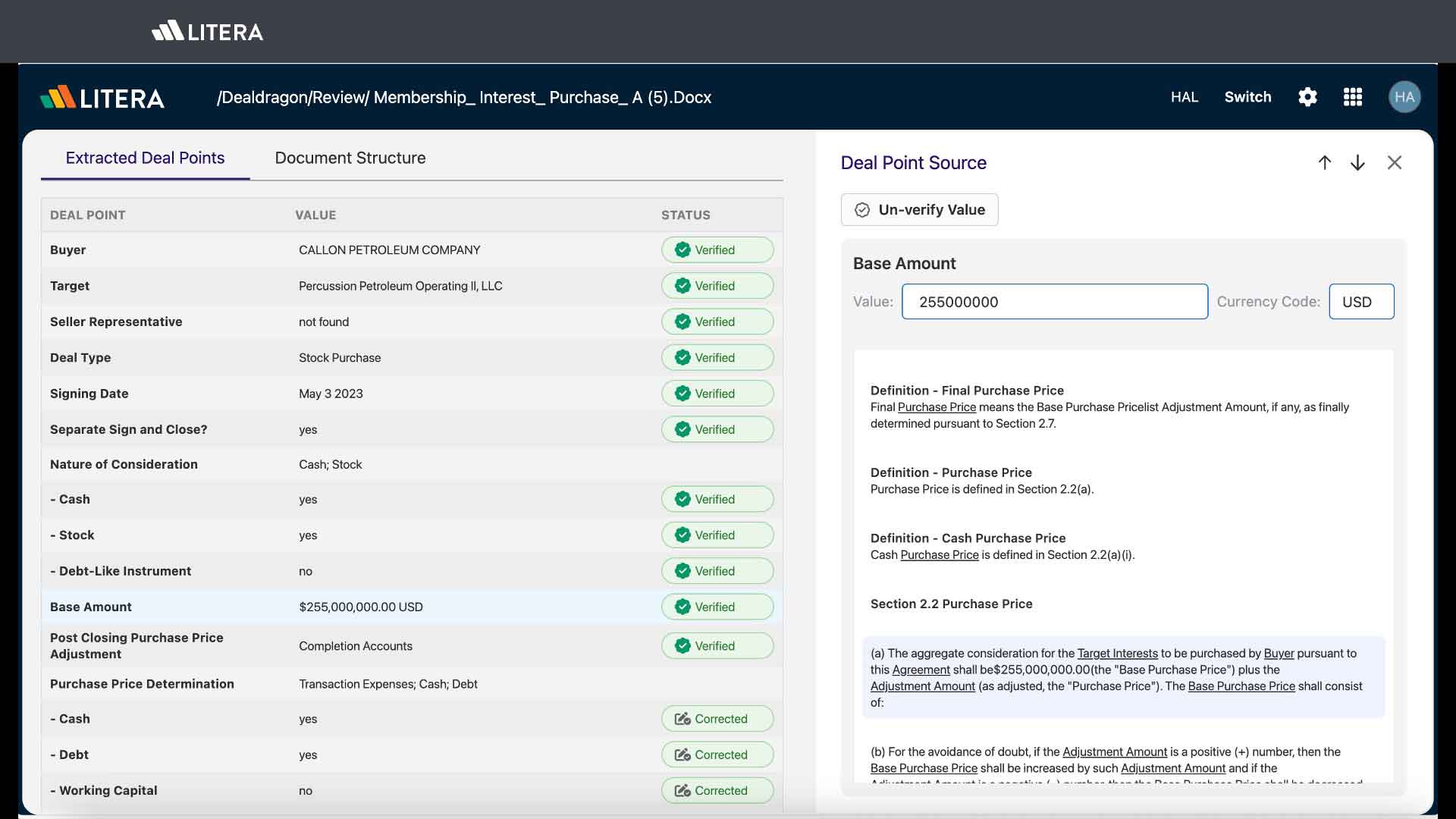
When GPT-4 first appeared on the scene, it really did seem like magic. Expectations ratcheted up to unrealistic levels. The hope was that we could just throw the tech at any undifferentiated pile of documents and have the data therein instantly structured, summarized, and synthesized in whatever manner would meet our needs. Those hopes have been dashed, thus far. But vestiges of the expectations remain, for good and ill.
How to Train Your Dragon
The consensus number one draft pick of the biggest GenAI disappointment among our roundtable participants is that GenAI alone does not solve their long-standing data and knowledge management challenges—rather, it makes the pain more acute.
GenAI has proven quite proficient at enriching data in a variety of ways [34]. But not proficient enough to overcome “data chaos” [35]. Rather, the pursuit of GenAI initiatives is creating a sense of urgency for enterprises to get their data in order [36]. More than the joy of having a new method to make use of data, businesses are feeling the hurt of their persistent data deficit as the GenAI revolution makes them more data oriented [37]. This includes the need to clean “dirty” legal data [38].


Dragon, for example, is fit for purpose. Dragon does not directly aid in drafting…yet. But if you point Dragon at done deals, it will populate a deal-points database like no tech that has come before. That is what Dragon was built to do. Dragon, however, was not designed to sift through every iteration and redline of deal documents in their pre-close, draft form. If you were to do something so silly as point Dragon at a folder with “Done Deal [Final]” and “Done Deal [Final Final]” and “Done Deal [Final Final Final] v.27”, its outputs would be very confused (unless you had an alternative aim, like tracking the evolution of a single deal).
Which seems extreme. As it should, especially in an areas like M&A where we are supposed to have a single source of truth, such as closing binders. But more than one presenter at our invite-only roundtables shared failed experiments of pointing GenAI at an undifferentiated mass of documents and not achieving the desired outcome.
For example, simply feeding an LLM corporate policies and the attendant attorney work product (e.g., advice emails related to the policy) does not in and of itself result in a functional employee-facing policy chatbot. And that’s only if the data is in an accessible format—as ediscovery nerds have long known, PowerPoint and OneNote are among the many not-so-accessible data storage formats.
Another common pre-GenAI pain point: many corporations have no idea where all their contracts are. GenAI does not alleviate that pain. It makes the pain worse because LLM-powered search of vector databases has opened new possibilities in contract analysis—but only after you locate and collect all those finalized contracts.
Many of the initial use cases for GenAI involve discrete data sets, like closing binders and collected contracts. Ediscovery is another prime example.
There is much that can be accomplished combining GenAI with a clean, discrete data set. But the work still needs to be done to construct a clean, discrete data set. With GenAI, the road to [thick-wrapper] product is long. And the road to implementation can be even longer due to the effort required to become AI ready.
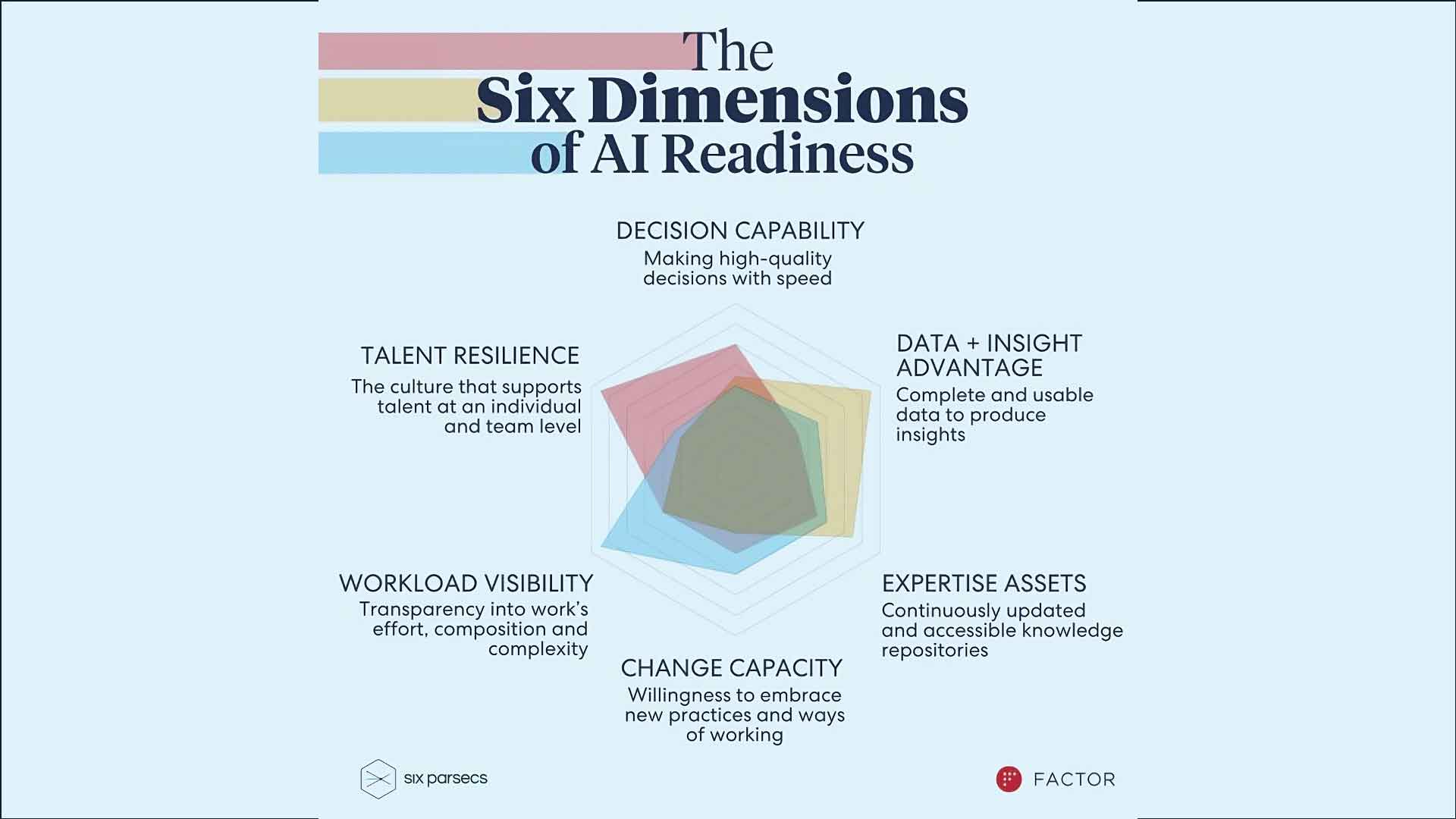
TAR Wars
Ediscovery was brought up by several presenters at the LexFusion events in New York, San Francisco, and London, including one prestigious firm sharing the results of rigorous testing—comparing GPT-4 with first-level reviewers on precision and recall. According to the firm’s results, GPT-4 outperformed the humans and hit benchmarks that exceeded the best-available technology-assisted review workflows (“TAR”).
Better. But still not perfect.
We’ve seen this movie before. Many times. The TAR Wars are entering their third decade, and the sequels are increasingly boring.
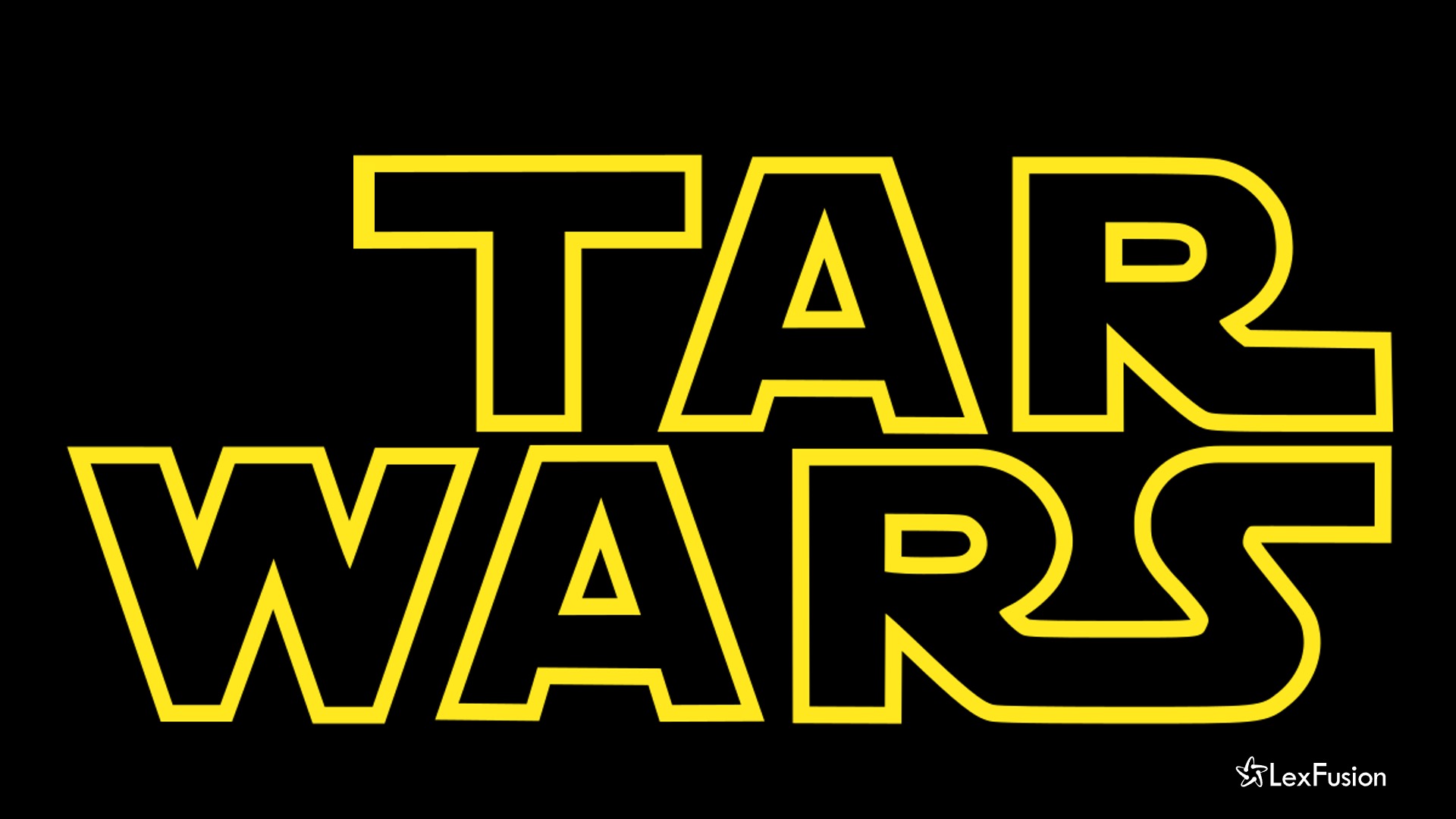
We’ve known since at least 2005 that “automated techniques can do a significantly more accurate and faster job of reviewing large volumes of electronic data for relevance, and at lower cost, than can a team of contract attorneys and paralegals.” [39] We have academic research finding “the myth that exhaustive manual review is the most effective – and therefore, the most defensible – approach to document review is strongly refuted. Technology-assisted review can (and does) yield more accurate results than exhaustive manual review, with much lower effort.” [40] We have confirming case law: “TAR has emerged as a far more accurate means of producing responsive ESI in discovery than manual human review of keyword searches.” [41]
And yet, because of FUD (fear, uncertainty, and doubt), the usage of TAR in ediscovery continues to lag [42, 43]. This, in part, is about people having a hard time trusting a black box. But it is also due to the abiding belief in humans as some infallible gold standard [44]:
It is not possible to discuss this issue without noting that there appears to be a myth that manual review by humans of large amounts of information is as accurate and complete as possible – perhaps even perfect – and constitutes the gold standard by which all searches should be measured.
Facts rarely change minds [45]. You tell lawyers the machine is 85% accurate, they identify that as unacceptable—because 100% is required. You explain to lawyers that humans are only 65% accurate, they simply don’t believe you even if your conclusion is supported by empirical evidence. Alternatively, they concede that, generally, humans perform worse than machines but contend that their special collection of humans are all outliers.
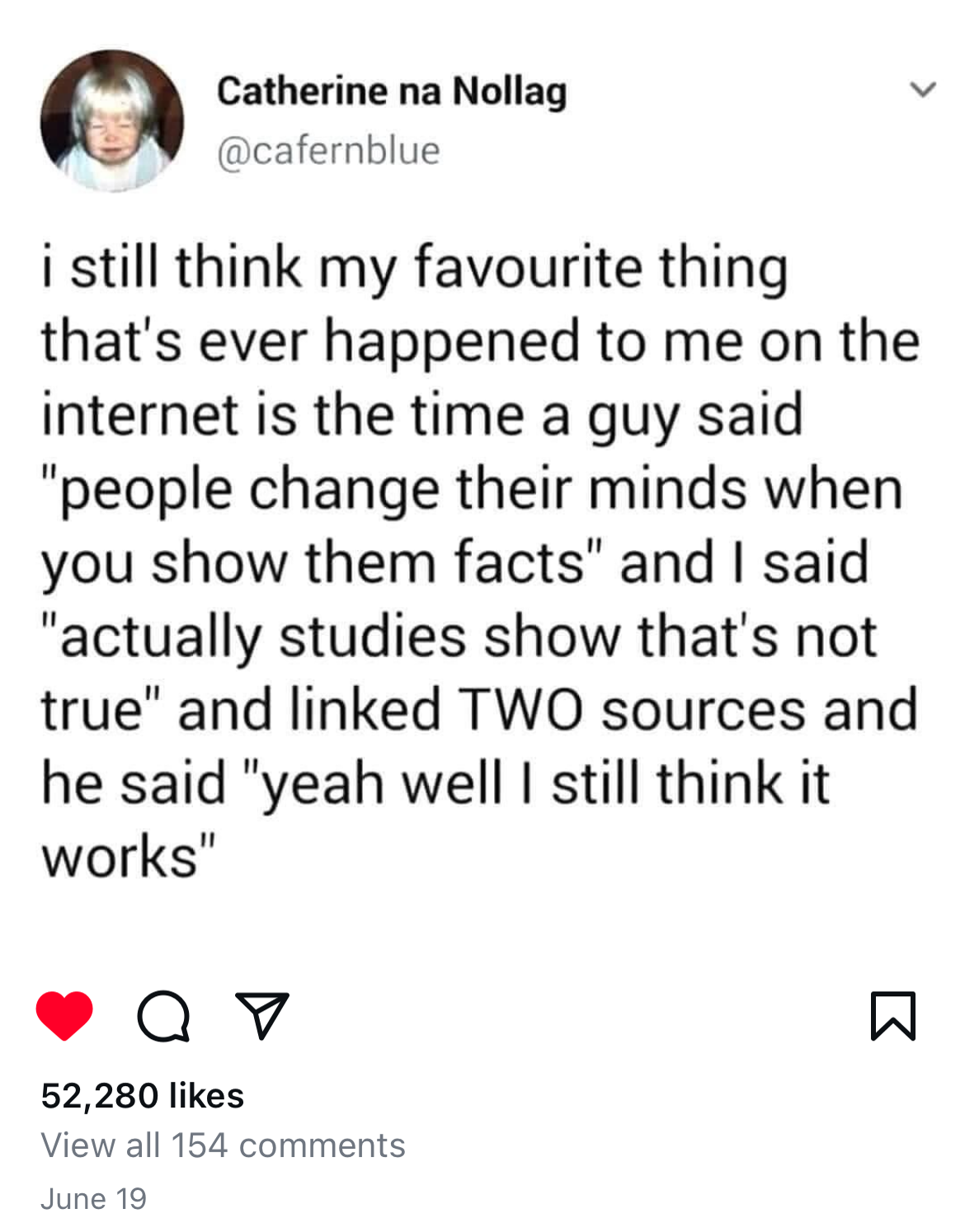
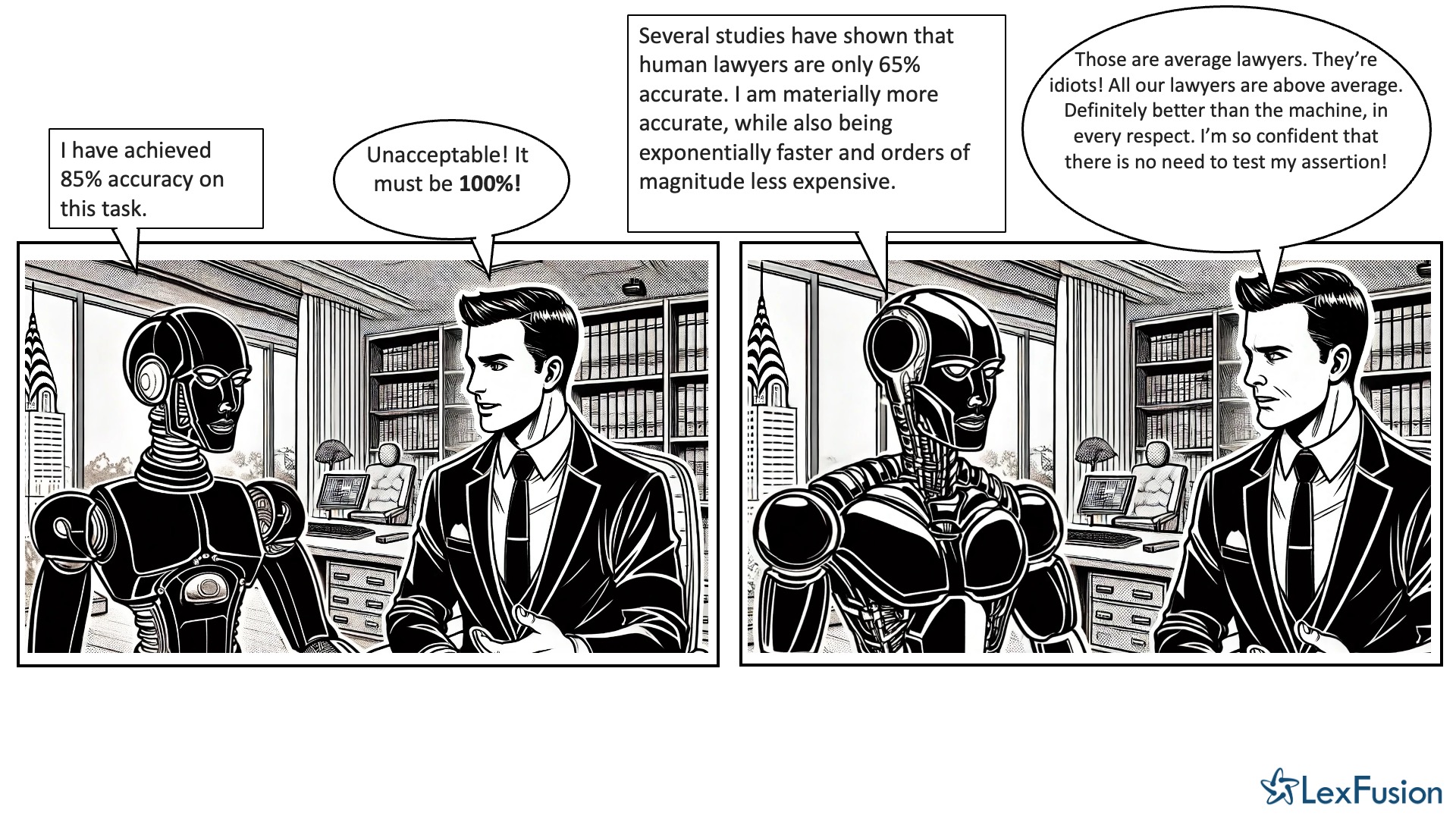
Given the preoccupation with perfection, hallucinations are, supposedly, an issue of immense concern to lawyers with respect to the use of GenAI [46]. But what if we told you that research has established that while GenAI will hallucinate even when performing a task as seemingly simple as summarization, humans hallucinate more when performing the same task—i.e., “LLM-generated summaries exhibit better factual consistency and fewer instances of extrinsic hallucinations.” [47]. Moreover, “LLM summaries are significantly preferred by the human evaluators.”
%20Dead.jpg)
Such findings keep coming [48] and are more than an abstract observation. One large law department presenting at our roundtables specifically cited their own experiments on GenAI outperforming humans-who-went-to-law-school at summarizing depositions—a task on which this law department currently spends significant sums. When asked by an audience member what the law department intended to do with this finding, no words were minced. For the time being, the law department was only enhancing their own understanding of where and how the new technology might be usefully deployed. But, soon enough, their understanding would evolve their expectations as to what they would, and would not, pay their law firms to do.
What is true of summaries, and ediscovery, is also true of contracts. In Better Call GPT, Comparing Large Language Models Against Lawyers, the researchers used senior lawyer judgment as a “ground truth” to benchmark both junior lawyer and legal contract reviewer efficacy at locating legal issues in contracts [49]. The study concluded, “advanced models match or exceed human accuracy in determining legal issues. In speed, LLMs complete reviews in mere seconds, eclipsing the hours required by their human counterparts. Cost-wise, LLMs operate at a fraction of the price, offering a staggering 99.97 percent reduction in cost over traditional methods.”
Better. Faster. Cheaper. And those are the raw models—not thick-wrapper applications engineered to purpose. Moreover, we’re still early days. The AI available today is the worst AI we will ever use—and it already surpasses human performance on many tasks. Using GenAI will be risky for businesses, including law firms, but nowhere near as risky as not using GenAI. Evaluation and judgment will be key for prudently balancing risks. Or you could just stick your head in the sand—which brings us to our generous friends at Carlton Fields.

No straw man required
Thankfully, there is no need to assault a straw man. We have volunteers. Carlton Fields raised their hands to be the standard bearer for tech-averse lawyers reduced to paroxysms at the notion of imperfect AI being additive to imperfect humans at some tasks lawyers currently perform.
The general counsel of Carlton Fields, Peter Winders, graciously provided us with a near-perfect specimen in Why Our Law Firm Bans Generative AI for Research and Writing [50]. Winders voiced publicly objections LexFusion encounters in private.

If the tool is working as described, predicting the most likely human response based on an enormous library of human works, then all it does is “hallucinate.” It predicts what a human would say, barely tethered by fact
….
What about a generative AI program trained only on a large library of only reliable material, such as the West system or the preserved research and output of a large law firm? This might cut down on the risk that the generative AI tool has scraped rubbish off the internet at large, but it will do nothing to stop its resort to the fabrication of cases.
We have no way to determine what the tool saw, what it missed, what it overlooked, what it misunderstood, the analogies it failed to make, what authorities were truly supportive or adverse based on a nuanced understanding of the facts of our own case and the facts of decided cases, what policies animated these cases, or what lines of analysis might have been left out of the query used for the search.
Of course, on its face, human-authored research presents the same issues. The human author of a research memo also doesn’t know what they missed, overlooked, misunderstood, etc. And then there is the rude reality that a study [51] of lawyer-authored briefs, filed publicly by law firms on behalf of their clients, found:
Nearly every brief we analyzed contained misspelled case names, miscited pages, and misquoted cases and statutes. Interestingly, about one third of the misquotes appear to be intentionally inaccurate…Most of the briefs in our analysis make the mistake of relying on precedent whose outcome supports the other side.

How then does Herr Winders baseline human performance to determine it remains superior to the machine at this particular task? He doesn’t. He conveniently presumes, prima facie, sapien superiority:
A generative AI tool can’t do any of these things because it can’t think. The narrative it generates is still a made-up answer created with algorithms, not with thought and judgment.
….
This isn’t like when a partner has to confirm that the cases a first-year associate cites are accurate. Associates are taught to think like lawyers, their drafts have thought and judgment behind them.
Ah, yes, the evergreen “they went to law school” fallback for ipso facto lawyer exceptionalism.
Yet playing against type, some denizens of the internet chose kindness and suggested that Mr. Winders’ headline Why Our Firm Bans Generative AI for Research and Writing was “click bait” and did not mean that the firm, in fact, bans GenAI for research and writing [52]. Instead, these sweet souls finely parsed Mr. Winders’ opening statement:
Our law firm has a policy forbidding our lawyers to use generative artificial intelligence to produce legal products such as briefs, motion arguments, and researched opinions.
The friendly reading was that Carlton Fields only forbids the use of GenAI to produce final work product—i.e., briefs, motions, and memos sent to clients or submitted to courts—rather than banning their lawyers from exercising judgment in taking advantage of new technological advances ‘in the production’ of said work product. We might call this the “Schwartz-Cohen Directive”: don’t blindly pass on machine-generated content as your own. Fair enough. There is, of course, the equally sensible “Junior Guardrail”: don’t blindly rely on junior-lawyer-generated content (a.k.a., Model Rule of Professional Conduct 5.1).
Whoever developed this policy apparently just doesn’t understand that generative AI cannot think
Everyone seems to think the risk of generative AI is “hallucinations.” Yes, that is an egregious risk. But nobody seems to get why those occur. They occur not because ChatGPT is playful or intentionally reckless, but because generative AI does not think.
And it doesn’t matter whether you restrict the data base used by ChatGPT – or similar tools –to WestLaw or the law firm’s own files. It still can’t understand what it reads, it can’t think, and it has no “idea” what it is saying about that data base in the ensuing narrative.
In our firm, we have adopted a policy that GenAI not be used at all in production of legal product, and we have viewed with dismay the pronouncements of some lawyers that GenAI is reformative rather than potentially destructive to quality lawyering.
Carlton Fields is not arguing that lawyers own whatever they put their name on (briefs, memos) and are responsible for that content regardless of provenance (i.e., their own research, research by another lawyer, research by an allied professional, machine-generated research, research found online, etc.). Carlton Fields is arguing that machine-generated research must be prohibited categorically. Apparently, GenAI is a tool so toxic that no lawyers, including their own, can be trusted to use it judiciously. Just like GPS should probably be banned because it is not completely accurate and, sometimes, those following it blindly end up driving into lakes.
For the sake of the argument, let’s assume that Carlton Fields does not prohibit digital caselaw research. Carlton Fields lawyers type in keywords. The system retrieves decisions containing those keywords and their synonyms. The system is not thinking (as Carlton Fields would seem to define it). The machine is merely pattern matching based on linguistic similarity.
Every lawyer encountered the limits of keywords in legal research & writing, if not sooner.

In theory, large language models, being large models of language, can augment the “and their synonyms” enhancement by expanding the search from keywords to concepts with the result being the retrieval of more relevant cases. More. Not necessarily all.
So what would Carlton Fields’ policy be if rigorous testing demonstrated that GenAI-augmented search retrieved more (not all) relevant cases than standard keyword searching? Presumably, still prohibit it (“it doesn’t matter whether you restrict the data base used by ChatGPT – or similar tools –to WestLaw or the law firm’s own files”). Yet, all we’ve done is graduate from one unthinking system to another with the latter yielding superior results. Beyond familiarity, why exactly are we banning the system with the superior results?
Note the bit about “rigorous testing.” The prior section on thin/thick-wrapper applications should make clear that LexFusion does not believe that every application of GenAI is automatically ready for primetime. It is entirely within bounds to test a GenAI application and determine the software is not sufficiently performant.
We agree with Wilson Sonsini’s David Wang that evaluation is mission critical [55]. We applaud Latham’s John Scrudato for advancing our collective understanding of how to test GenAI output [56]. We encourage everyone to read Jackson Walkers Greg Lambert’s analysis of the controversial Stanford study finding shortcomings in GenAI-enabled research platforms [57]. But we also remind you that previous studies have identified similarly problematic error rates from the professional human editors at Westlaw, Lexis, and Bloomberg Law [58].

There is an adage that it is AI until we know how it works, then it is just software. That’s drivel. A minuscule percentage of users comprehend how any of their software works. Few of us understand what is happening under the hood. Not just transformer-based neural nets and word vectors (foundations of GenAI), but the underlying ones and zeroes (binary code), as well as the attendant electrical currents and logic gates (what the binary code is dictating).
What is actually true is that it is scary until we know it works, then it is what is. Indeed, one amusing, and telling, definition of “technology” is “everything that doesn’t work yet.” [59].

Do you consider an elevator to be technology? How many of us actually understand the actual mechanics?
Elevators became essentially self-driving a few years after their introduction. Yet elevator operators did not begin to disappear for almost 50 years—until they went on strike (oops!) [60]. It was not just that riders started pushing the buttons themselves. The shift also occurred because elevator manufacturers began introducing user-friendly improvements, like Help buttons and emergency phones, enhancing user comfort levels by signaling that while something might still go wrong, it couldn’t go too wrong.
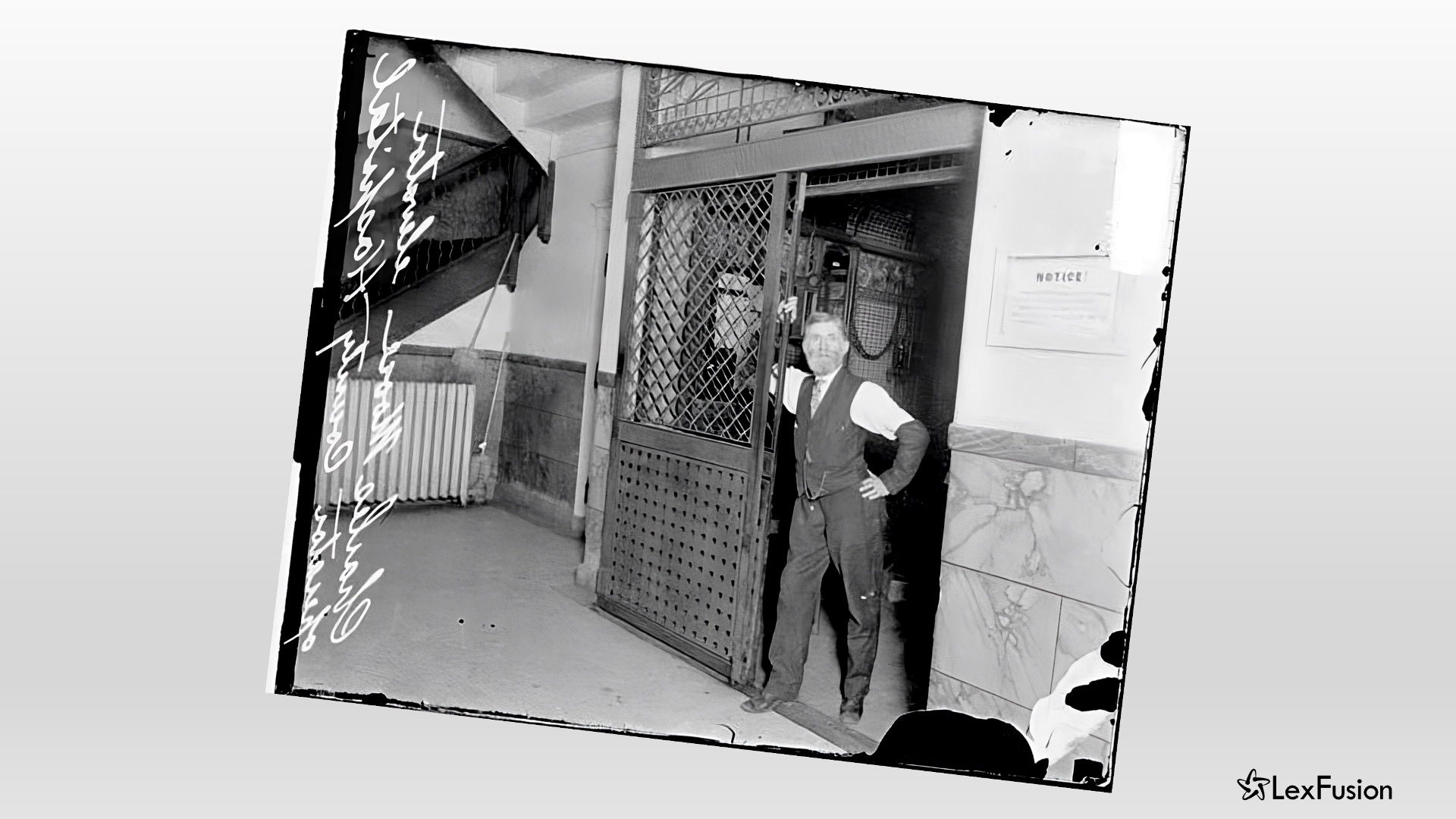
Elevators still malfunction. But this is almost always an inconvenience, not a tragedy. On net, elevators, while imperfect, are superior to stairs for many use cases, even if mechanization induces some level of laziness with obvious downsides. Modern skyscrapers would simply not be functional spaces without the elevator—it is a form of automation that enabled unprecedented physical scale. Mr. Widner, however, would have probably called for elevators to be banned because the elevators don’t ‘climb,’ or some other anthropomorphized inanity that distracts from legitimate questions—on net, does the elevator cost-effectively accomplish its purpose? Is the risk of malfunction, in terms of frequency and severity, worth the benefits?
We must ask questions. But our questions should be good. The answers are important for determining the acceptable level of automation. Acceptability being an interplay between performance and comfort. Comfort matters. But not all forms of discomfort should be afforded equal levels of deference.
For example, a recent study found that incorporating AI into reading EKG results “reduced overall deaths among high-risk patients by 31 percent.” [61] What if a doctor who was responsible for your care, or the care of a loved one, refused to take advantage of this superior performance because the AI wasn’t thinking? It would be laughable if it were not life threatening. All the monitoring system is doing is more accurately focusing finite human attention (just like better case retrieval).
Yet, for now, most people would be far less comfortable if the AI’s purview expanded to unsupervised intervention—with the AI making, and actioning, treatment decisions without a doctor in the loop (akin to the unreviewed, AI-generated brief). Which does not mean we’ll never be willing to rely on the machine (after all, we rely on the EKG; as we rely on pacemakers, etc.). But it will require more evidence of superior performance for us to reach the requisite level of comfort given the severity of the downside risks.
Rank versus Right Skepticism
Please do not let our low opinion of Mr. Winders’ lack of factuality—in his piece lamenting GenAI’s lack of factuality—suggest we are immediately dismissive of all GenAI skepticism. In many areas, more skepticism is warranted.
For a fabulous piece of skepticism, we commend GenAI and commercial contracting: what’s the point? from Alex Hamilton, CEO of Radiant Law [62].
Alex and Casey long ago made a public wager about the impact GenAI will have on contracting [63]. Alex proposed a bet that GenAI will not replace more than 5% of what lawyers currently do in the contract process within 5 years—4 years are now remaining. Casey gladly took the other side. As Casey has shared elsewhere, he is more worried about losing due to the timing, rather than the magnitude, of the impact. Again, Amara’s Law is that technology underdelivers relative to expectations in the short run even though it has far more impact than anticipated in the long run.
Alex and Casey agree more than they disagree. Alex and Casey agree:
- Much of what organizations are looking to accomplish with GenAI has long been possible through more straightforward standardization + automation approaches; it is just that few were doing the requisite foundational work, including the hard work of behavioral change
- GenAI is not magic; it does not eliminate all the complementary work many organizations have long avoided
- Many market participants are confusing means for ends, elevating GenAI as a solution in search of a problem
Casey and Alex’s points of disagreement are not really technical in nature. The diffusion of innovations is, after all, primarily a social process [64]. Casey is betting that the broader business implications of GenAI serve as an accelerant of pre-existing structural trends that ratchet up automation expectations.
For Alex, GenAI is TAR, which remains in relatively modest use after three decades. For Casey, GenAI is email, which required only a few years to become the bane of our existence.
Cassandra@prophet.com. Back in the halcyon, Hotmail days, lawyers were presented with email and immediately balked—email was, unquestionably, a data-security nightmare. Lawyers were 100% correct. Lawyers thought pointing out the very real (and realized) risks of email would result in enterprises not using email. Turns out, the upsides of instant but asynchronous communication outweighed the downsides.
This is obvious, in retrospect. At the time, however, it was apostasy.
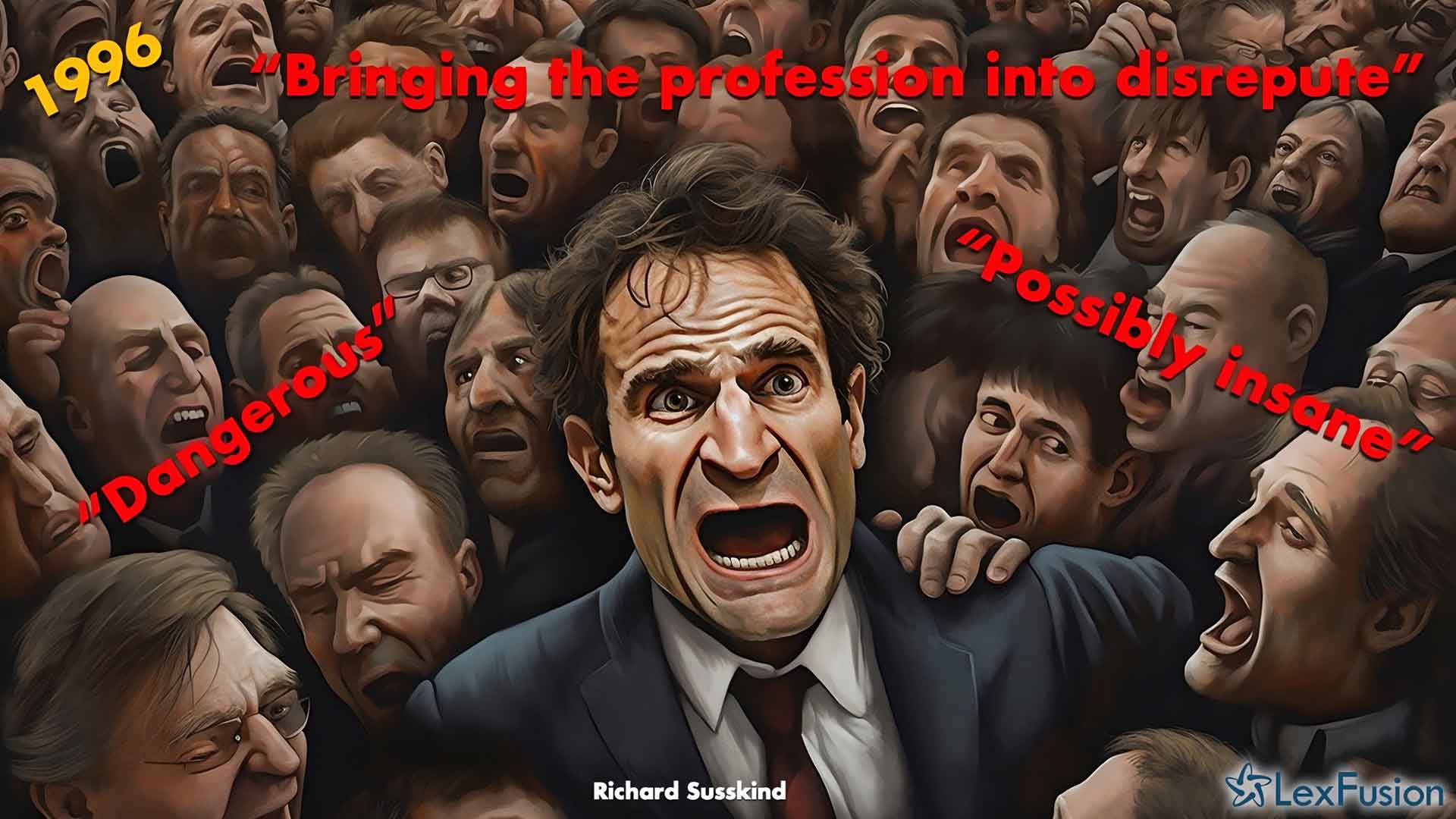
In 1996,, when WYSIWYG interfaces had made email broadly accessible, the legal futurist Richard Susskind was labeled “dangerous” and “possibly insane” because he had the audacity to predict email would become the dominant form of communication between lawyers and clients [65]. One bar association even took up a motion to prohibit Susskind from speaking in public because he was “bringing the profession into disrepute.” Within a few years, the legal industry was suffering from an acute Crackberry addiction. Even though Blackberries disappeared, the email epidemic still rages unchecked.
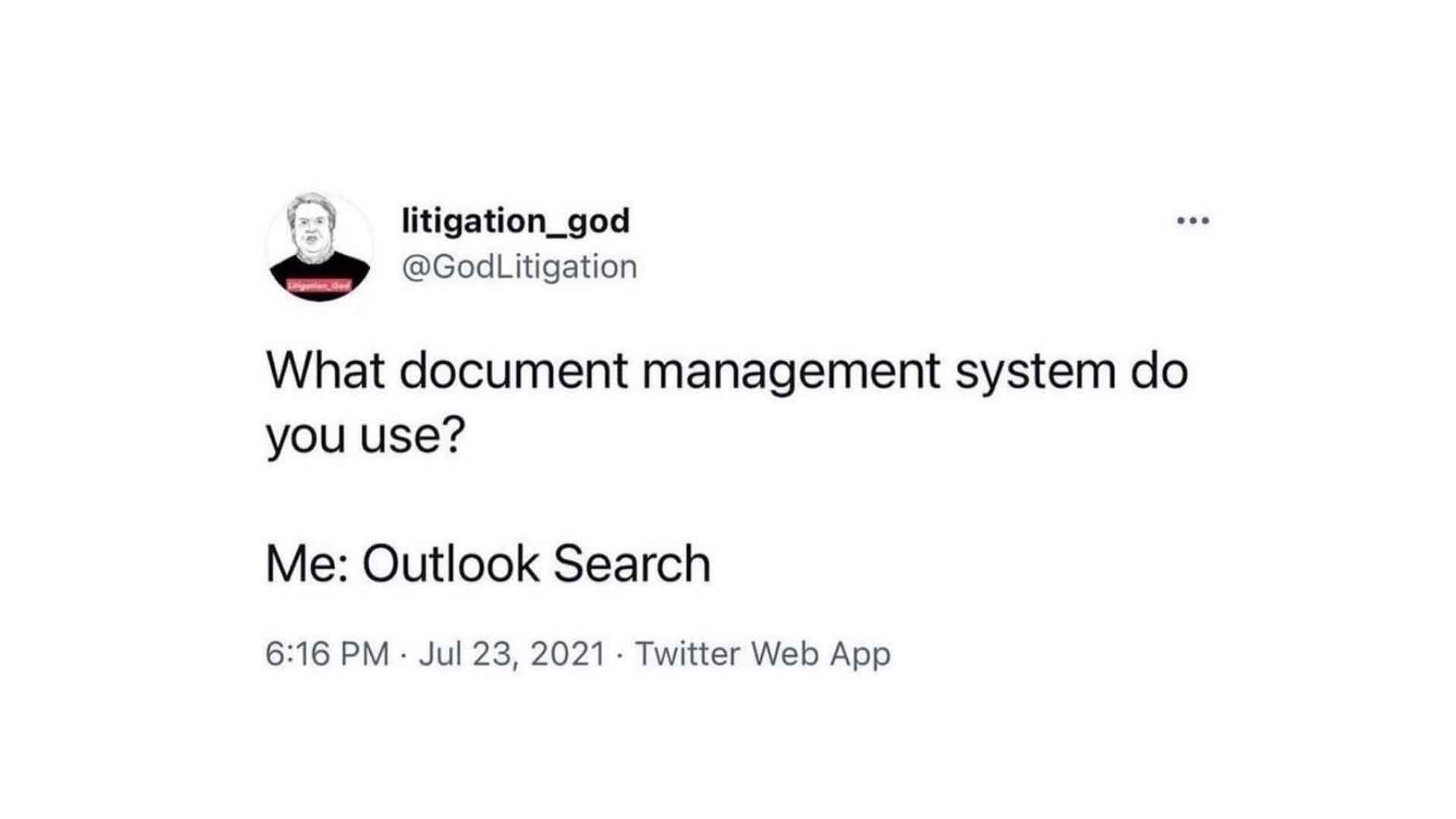
Vis-à-vis the legal market, Susskind was Cassandra, an accurate seer of the near future, doomed to not being believed until that future had already arrived. Vis-à-vis corporate clients, lawyers were the Cassandras, prophesizing that email would become a constant source of extreme pain—yet being mostly ignored despite being completely correct.
A primary difference between email (ubiquitous) and TAR (underutilized) is that the former is a general purpose technology whereas the latter is mostly limited to legal use cases [66].
The order of operations was that corporations adopted email, over lawyer objections. Inside and outside counsel then had no choice but to get on board. With TAR, by contrast, lawyers themselves have been able to throttle the rate of adoption.

In short, we’re saying the lawyers are not in charge here.
Great Expectations
GenAI is general commercial tech. Enterprises are pushing forward with GenAI initiatives [67]. Most are still in the experimental phase [68]. Enterprises are not AI ready [69]. They are learning that doing GenAI well is hard [70]. Widespread adoption will take time [71], and require complementary investments [72].
While AI readiness, like the tech itself, is still early days, the flurry of activity reflects the ratcheting up of expectations. One can be skeptical about the tech. But it is a mistake to dismiss the expectation shift, even if you consider it grounded in flawed premises. Current expectations have a profound impact on future reality even if current expectations are misaligned with current reality. Again, the diffusion of innovations is primarily a social process.
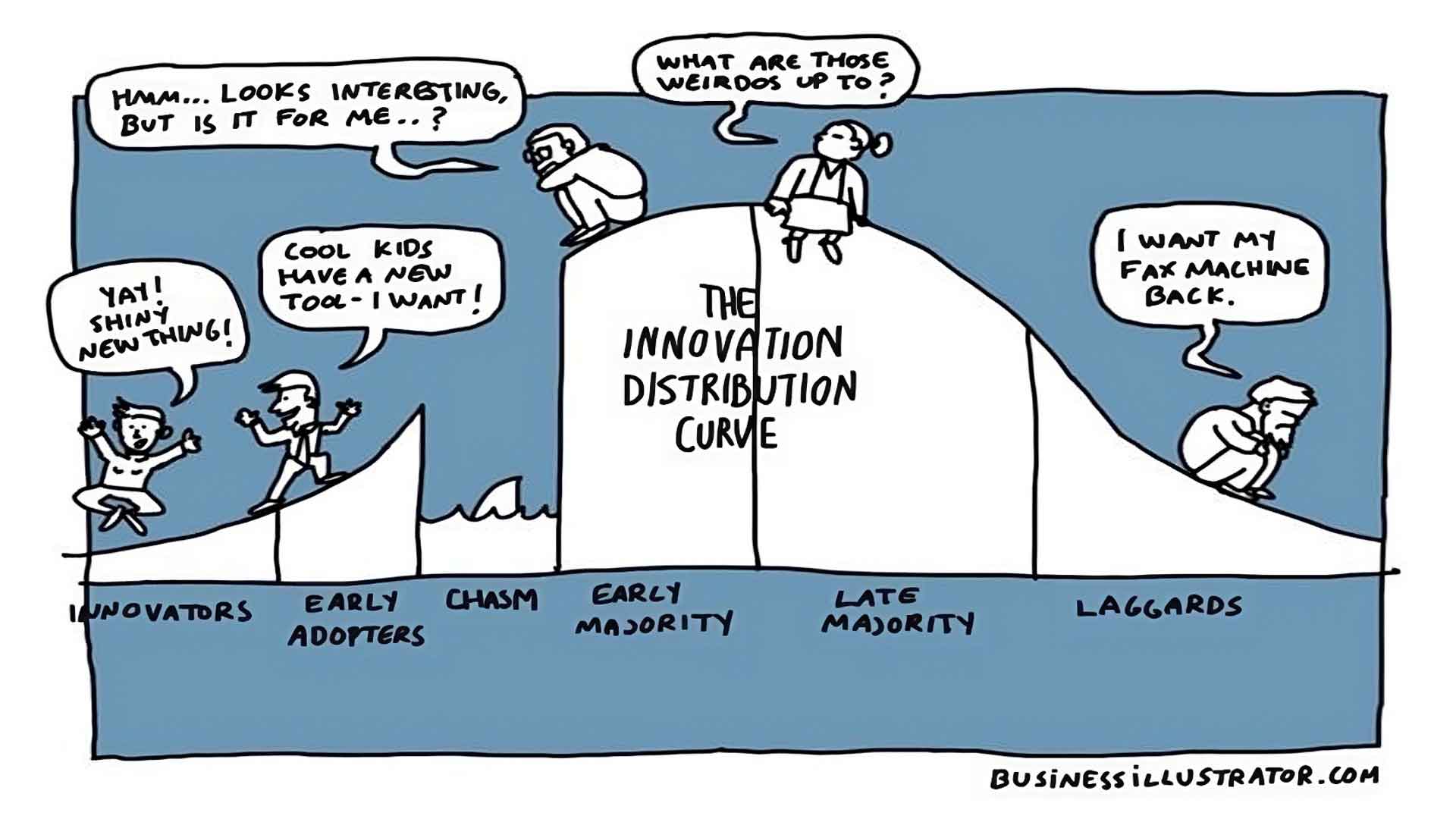
There is considerable merit to argument that the automation/efficiency gains many are hoping will be produced by AI magic have long been available through the rigorous application of existing technologies and methodologies. But such grievances are really about the level of motivation required to overcome inertia. GenAI has proven a motivation multiplier, a tipping point precipitating a period of punctuated equilibrium.

For example, even if GenAI turns out to be useless vaporware, the fact still remains that enterprises are exploring GenAI use cases in earnest. These explorations create net new work, in novel areas, for law departments. It is also leading to net new complexity in the form of new regulations. The advisory work is real. The regulations are real. Regardless of whether GenAI itself is empty hype.
Work requires resources. But the net new work is not accompanied by net new resources. Instead, it is more work with fewer resources.
One survey found, unsurprisingly, that 100% of CEOs planned to make significant investments in GenAI projects. More tellingly, the same survey found that 66% of those CEOs were planning on funding these GenAI projects by raiding existing budgets [73]. That is, while workloads are increasing in unplanned ways, resource constraints are also increasing in unplanned ways [74]. Law departments are, in turn, cascading these shifting resource-to-output expectations down to law firms [75].
In general, enterprises have increased their emphasis on enhancing productivity over adding personnel—capex over opex. This has long been the direction of travel. The pace is simply faster now because of the way GenAI has recalibrated expectations. That GenAI might not always be the most immediate, nor even best, option for addressing ratcheting automation expectations is somewhat besides the point. The expectation shift is real, and already responsible for real-world impact.
Jevons Paradox
The expected real-world impacts do not include the end of lawyers.
When gas is cheap, people drive more, and the aggregate usage of gasoline increases. This counterintuitive but commonly observed phenomenon is known as Jevons Paradox [76]. Its technological corollary is known as the Automation Paradox [77].
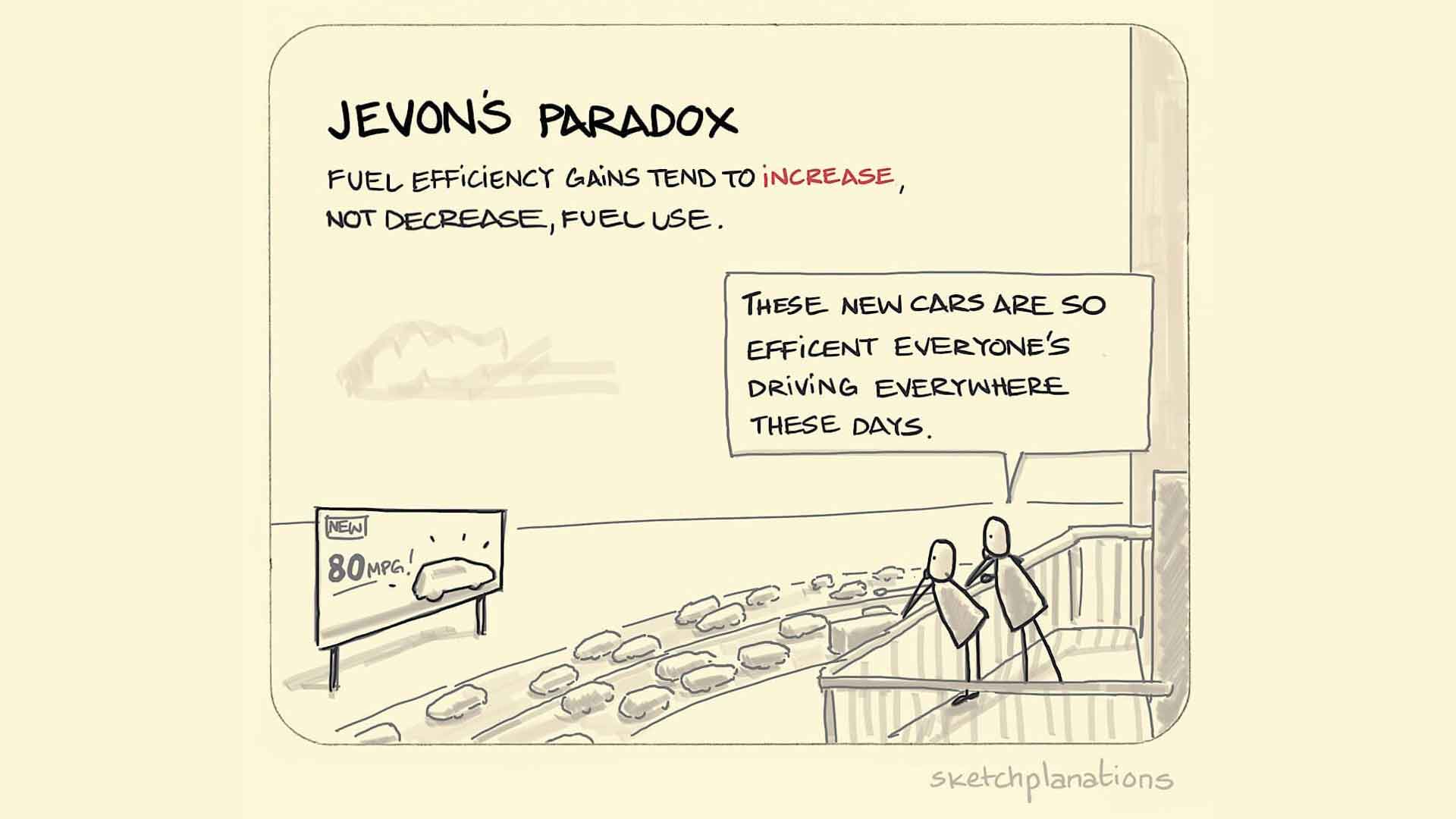
For example, ATMs (literally, automated teller machines) reduced the number of bank tellers required per bank branch. But, for decades after their introduction, ATMs did not reduce total teller employment because, with lower staffing requirements, ATMs enabled the number of branches to proliferate [78].
Excel reduced the number of bookkeepers and clerks. But it increased the number of accountants, auditors, analysts, and financial managers by an even great number [79]. Total work went up, not down. Work did not disappear. Work changed.

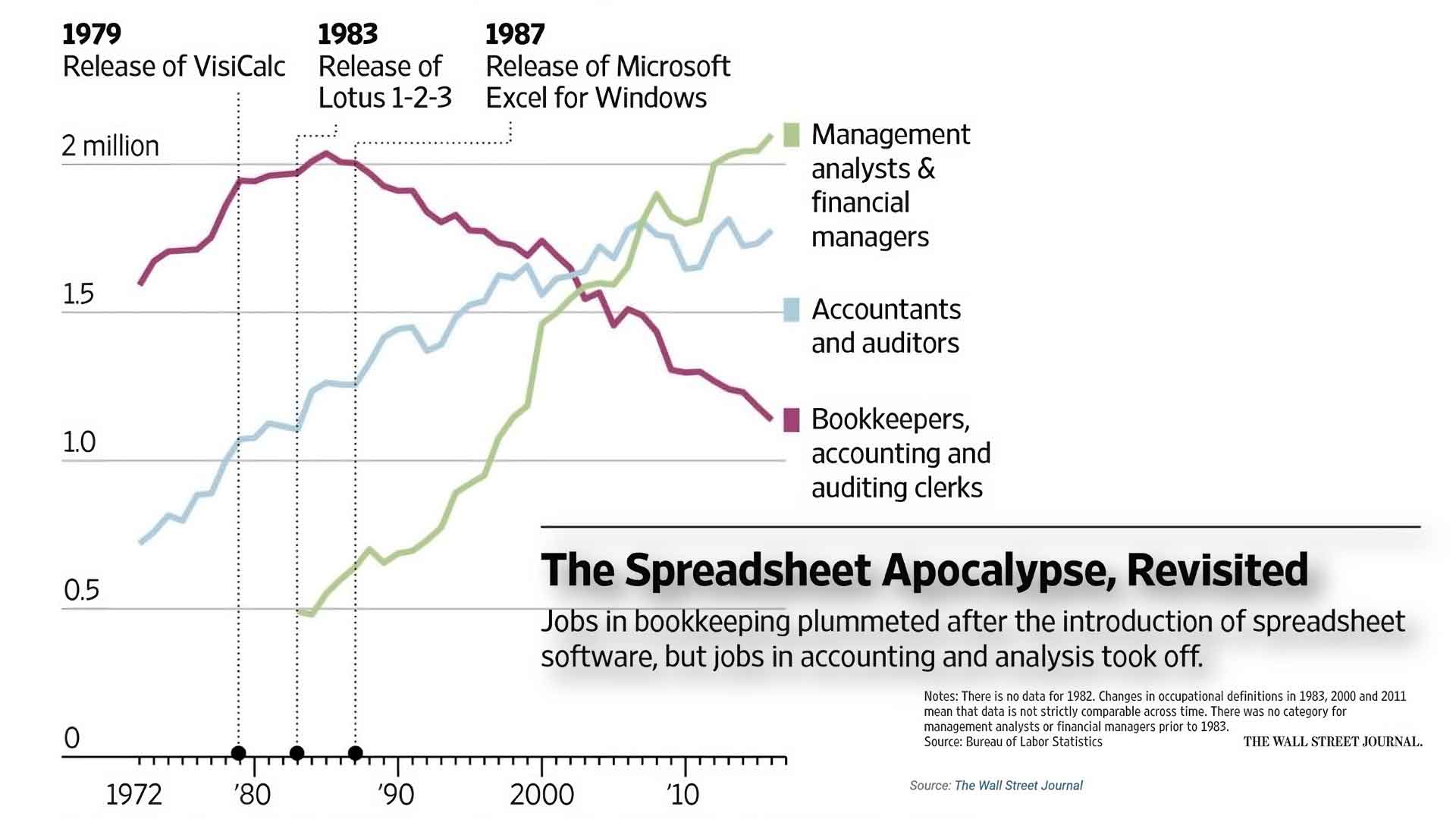
Email reduced the cost, and increased the speed of, communication. We now communicate more, for good and ill. The net impact is more work.
GenAI reduces the cost and increases the speed of knowledge-intensive tasks—both production and consumption [80]. At LexFusion, we expect this will result in increased knowledge work and, with it, the demand for well-trained lawyers.
Litigation is an obvious example. New enterprise activities + new regulations create new areas for investigations, regulatory interventions, and lawsuits. Meanwhile, the new technology enables regulators, plaintiff attorneys, and consumers to credibly maintain more actions. When the cost of war (litigation) goes down, there are more wars (litigation)—and you need more generals (lawyers).
Yet it is a mistake to focus on the use of GenAI by lawyers and the opposing parties that keep lawyers gainfully employed. GenAI in legal is a second-order concern. Primarily, the use of GenAI by corporate clients is what will increase the volume, velocity, and complexity of legal work. Lawyers’ immediate emphasis should be on advising the business on the proper use of GenAI. Lawyers’ medium-term preparation should be directed toward keeping pace with the all the new work generated by the business’s proper, and improper, use of GenAI. Law departments and law firms will need to adopt new tools and new ways of working simply to fall behind more slowly.
The best analysis of the magnitude of the impact GenAI will have on the corporate legal wallet comes from Jae Um of Six Parsecs and Ed Sohn of Factor (Ed is the man behind the curtain of Factor’s AI Sense Collective)—both of whom presented at the LexFusion roundtables. Their two-part Sense and Sensibility could not be more highly recommended [81].
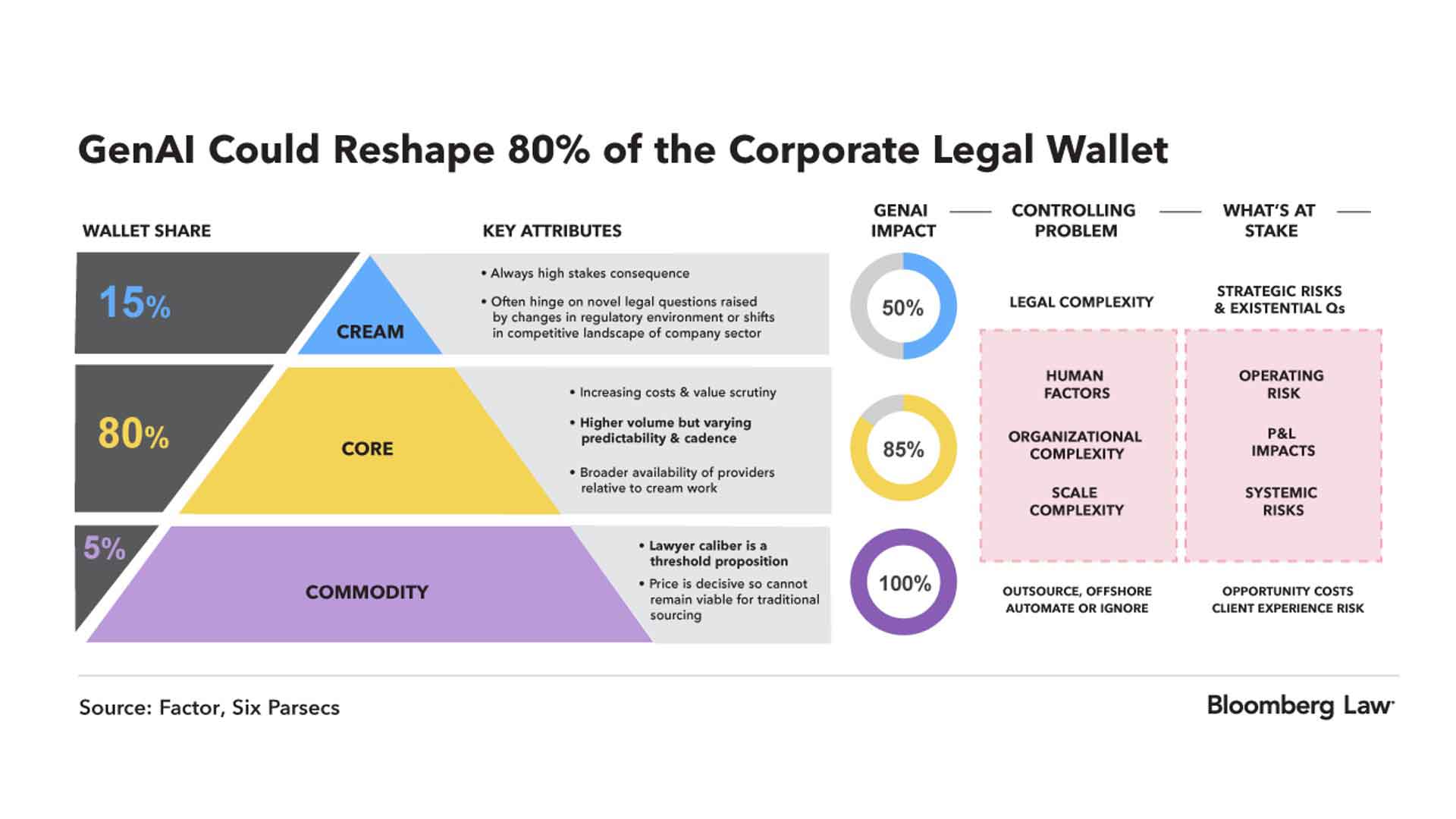
We are on the precipice of a phase shift. There is no holding back the strange AI tide [82]. And, yes, this will certainly involve pedestrian dynamics, like law departments expecting cost savings from their law firms. But it is far more elemental than that.
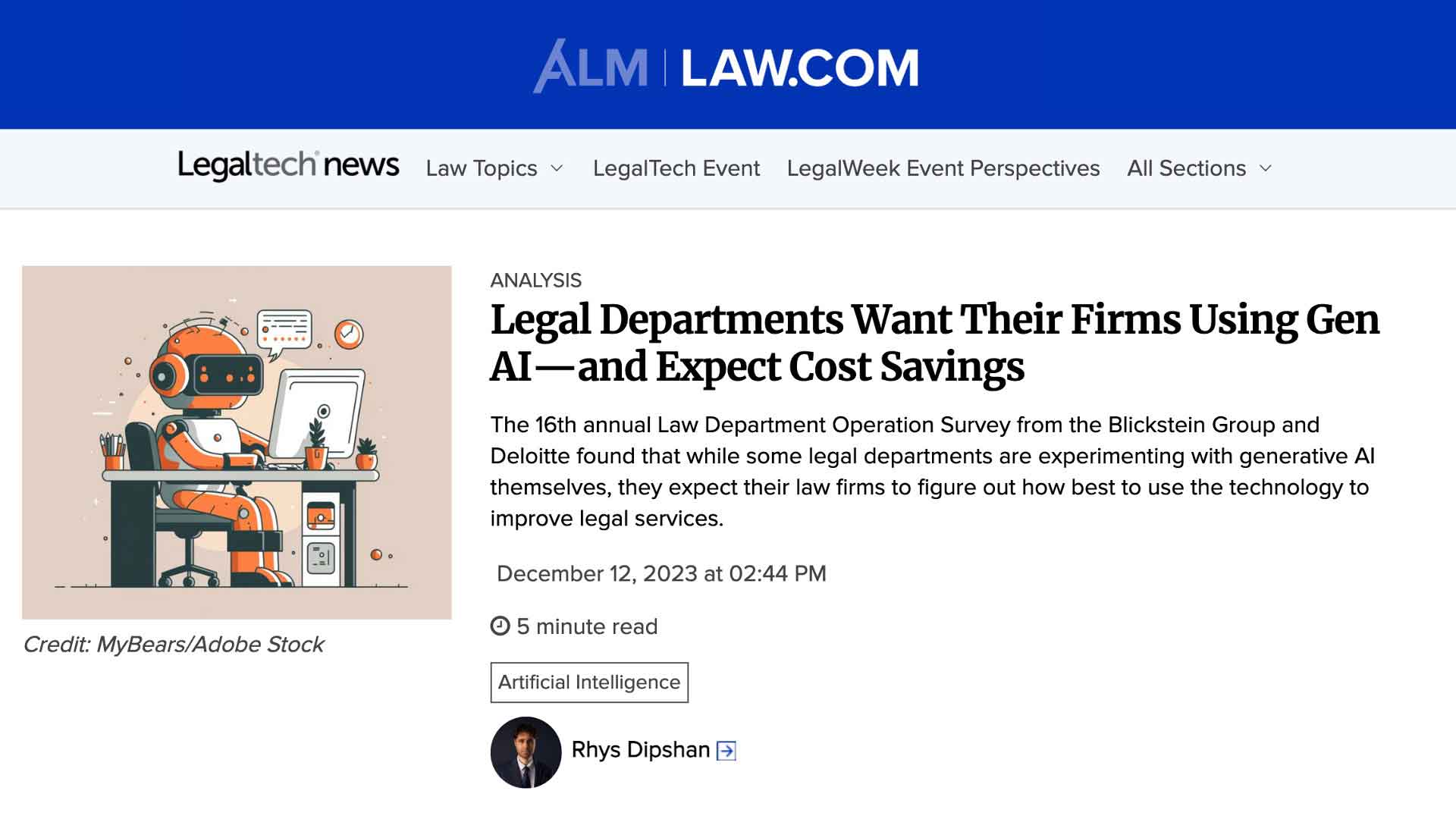
Consider our Dragon example. Today, a robust deal-points database is a competitive differentiator for a small subset of law firms because the databases are so expensive (mainly in terms of human labor). Dragon materially lowers the barrier to entry. But Dragon does not make a deal-points database free.
From the perspective of vast swathes of the market, Dragon will be a net new cost because, previously, their expenditures were zero. Staying competitive demands new attention costs in terms of evaluation, implementation, adoption, and operation. Staying competitive demands new fiscal outlays for the product itself, as well as the opportunity cost of the nonbillable time spent validating the pre-populated surveys—15 hours to 15 minutes is a magnificent reduction in labor intensity, but most firms were allocating 0 hours, meaning the 15 minutes represent an incremental cost.
Dragon, however, has changed the stakes. Firms will now be at a more significant competitive disadvantage if they choose to abstain. The lower barrier to entry shifts a robust deal-points database from being a competitive differentiator to a competitive necessity. Either ante up or fold.
Meanwhile, those few firms that already had the will and wherewithal to build a competitive advantage in the form of a robust deal-points database will witness their advantage shrink. The fact of having a database will no longer serve as a differentiator. They will need the best database: more deals, more fields, better discipline, better analytics, and better integration of the information into their pitches, negotiations, and drafting. Competition will narrow and intensify.
While, understandably, our default is to analyze law firms with respect to their role in the legal value chain, it is often helpful to remember that law firms are independent businesses navigating a hyper competitive market. Every enterprise will face tougher variants of the Dragon decision: new options, new costs, and new work, amidst fiercer competition.
This will not all go well. There will be all manner of innovation theater, delivering a bolus dose of unfunded mandates, unreasonable time horizons, and impossible expectations.
Things will get harder
More work. More complexity. Flat or declining budgets. Headcount constraints. What could possibly go wrong?
So much. Much can go wrong. Much will go wrong.
The parade of horribles has already commenced.
And while ‘progress’ seems inevitable, if frequently premature, the cautionary tales will only accumulate.
Well-trained lawyers will be more essential than ever to navigate an increasingly complex business operating environment, as well as clean up the messes when that navigation inevitably goes astray. Heightened demand will be paired with heightened expectations of how demand should be met, including decreased legal budgets and headcount on a relative basis. Innovation will be imperative.
This is not exactly fair.
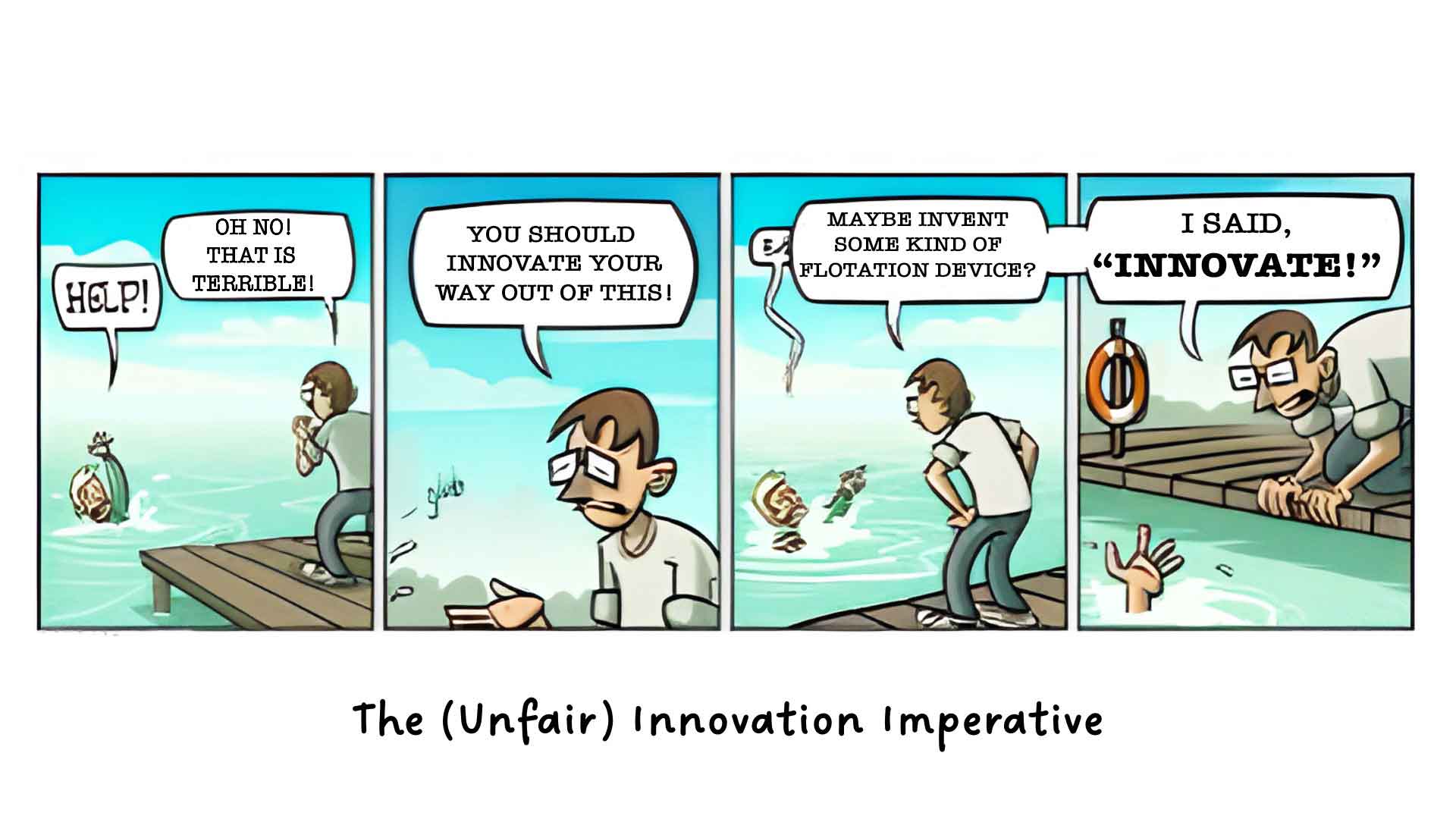
Law departments will need to solve for scale despite never being built to do so—i.e., shrink the expanding gap between growing business needs and flat legal resources.
These folks are already burned out. They already have more substantive work than they can handle. How are they supposed to modernize the plane midflight without ever landing it for repairs? How are they to overcome the choice overload of a legal technology ecosystem overrun with thin-wrapper applications (HINT: call LexFusion), let alone carve out time for process re-engineering, knowledge management, and all the other foundational work of successful tech-centric improvement initiatives?
We’ll survive and, most likely, thrive
Number crunchers flourished after the introduction of automated spreadsheets, despite their work being totally transformed. Email enabled enterprises to achieve unprecedented speed and scale despite being a data-security nightmare—just as elevators, quite literally, allowed buildings to reach new heights despite increasing the odds of plummeting to one’s death. GPS profoundly changed how we navigate physical space, despite atrophying our ability to orient and sometimes directing us to drive into lakes.
We’re playing with fire. Fire can burn. Fire can destroy. But fire is also a source of light, heat, clean water, and cooked food.
What’s coming will be hard, not impossible. These are the big leagues. It is supposed to be hard. Legal professionals are expert at doing the hard things well.
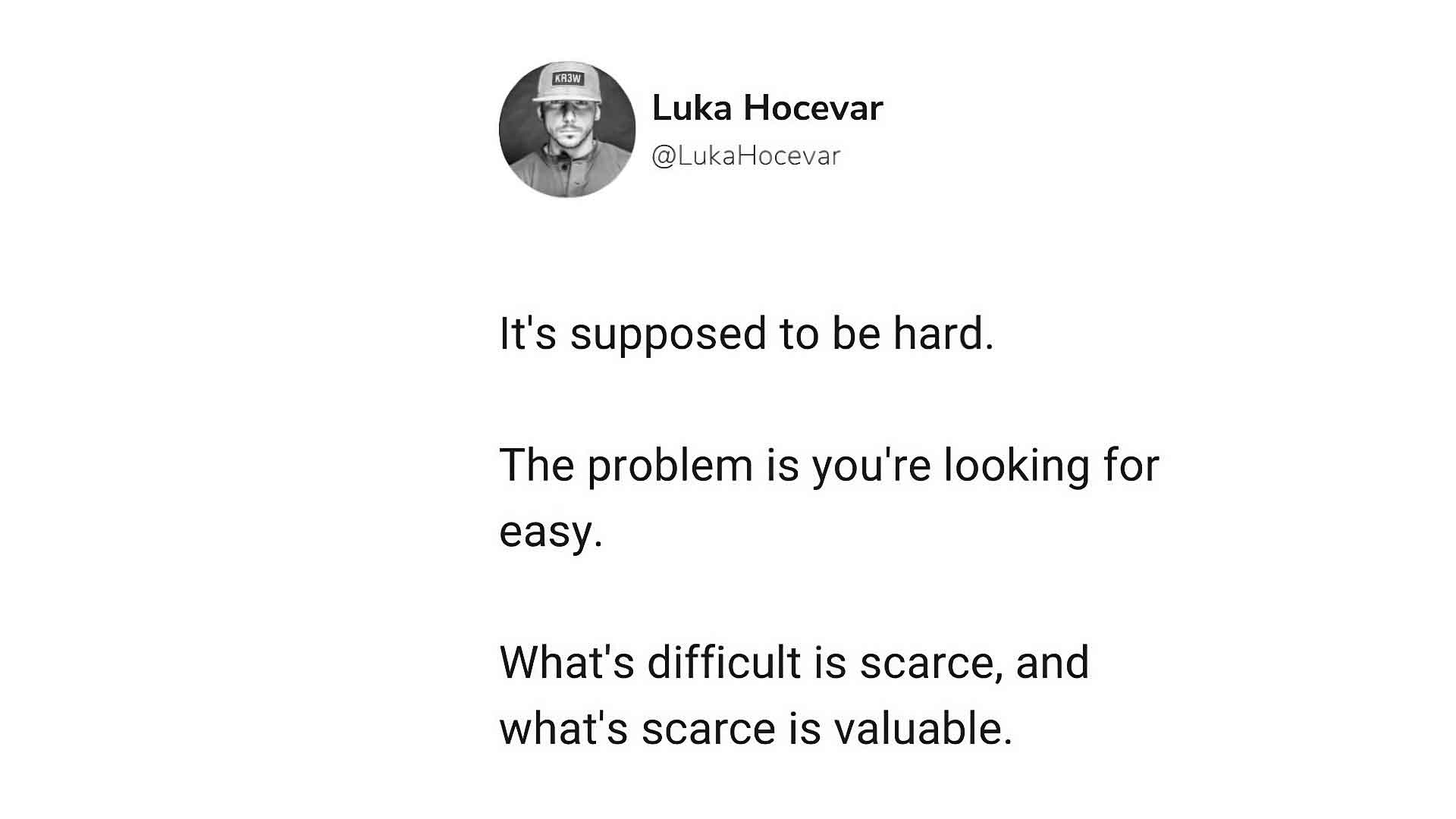
While GenAI’s dominance of the collective conversation may already feel interminable, we’re still only at the beginning. We remain early days, relatively speaking. Legitimate excitement. Valid concerns. Considerable uncertainty. Well-earned annoyance. Unprecedently fast (relative to prior general purpose technologies). But seems slow (relative to expectations). So much promise. Yet way too much hype. Change is coming, but not all for the good. Immense pressure to act. Not enough time to think. With legal professionals bowing to no one in our disappointment and doubt [83].
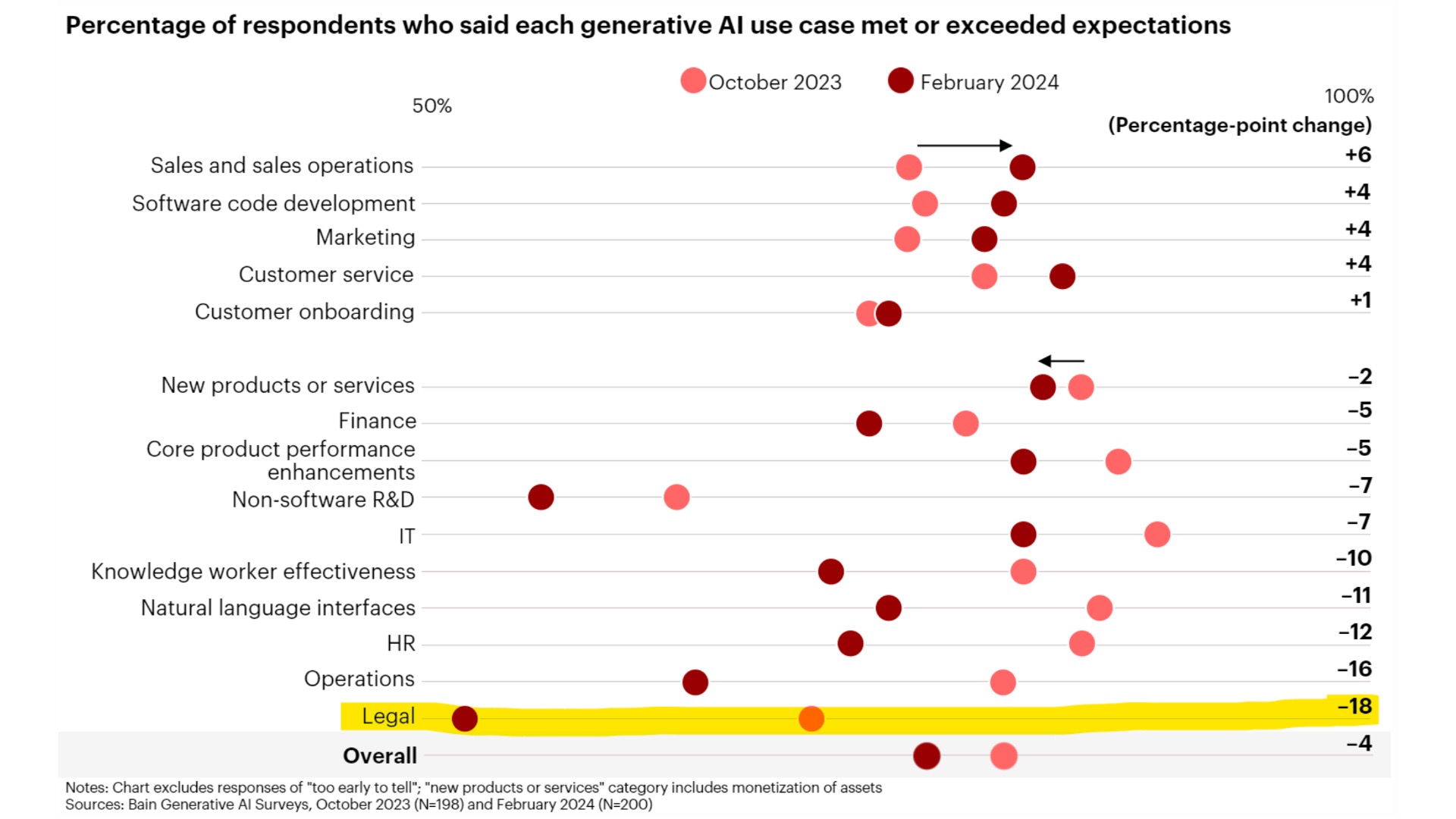
If you came here for definitive answers, sorry to disappoint. If you came here to frame better questions, LexFusion hopes to have been of some service. There are few Easy Buttons, and even fewer easy answers.
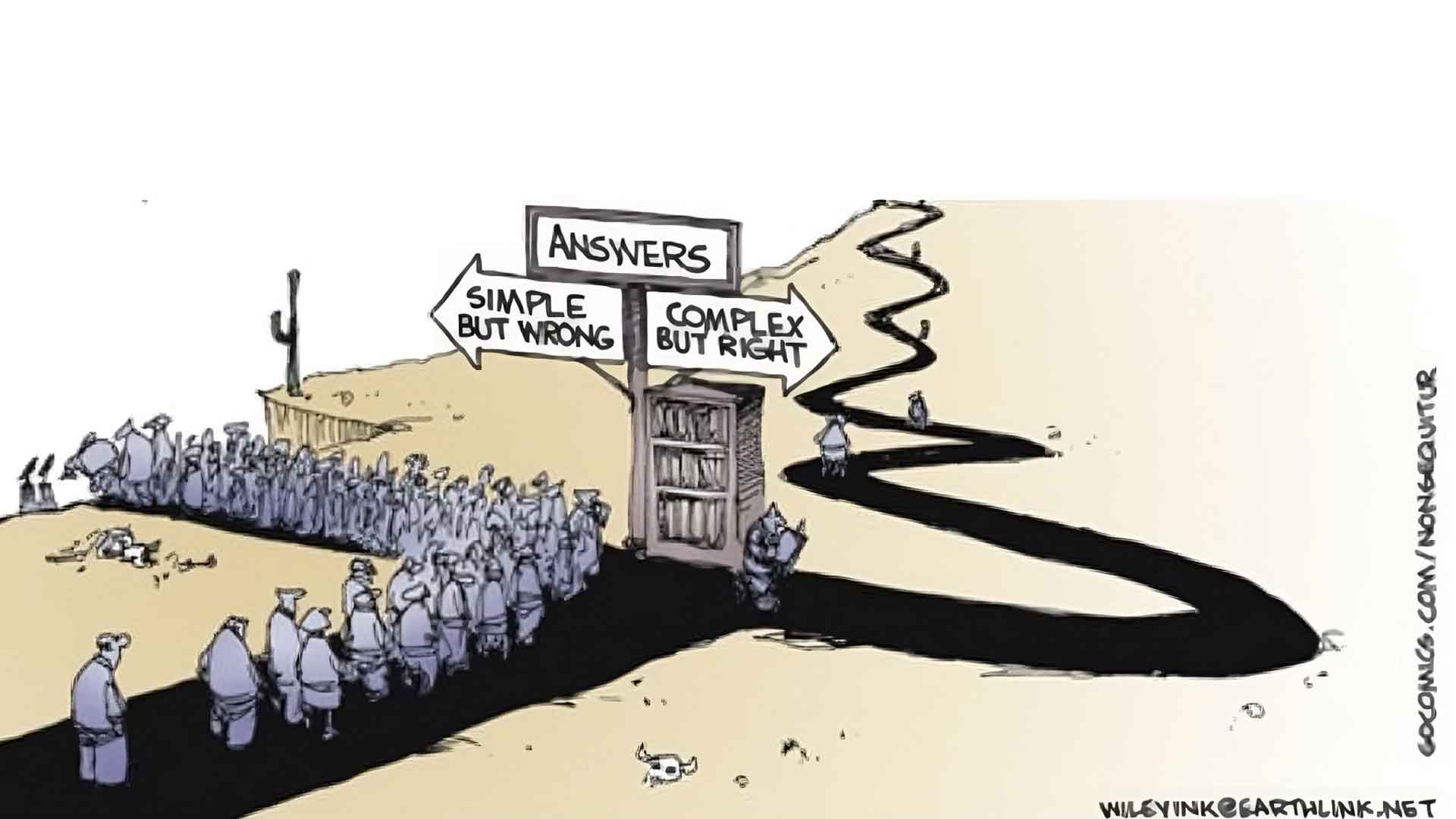
If you’ve visited our content library [84], you know we do our best to stay informed, and be informative. Yet while the foregoing is long, this topic is so vast that it feels like we’ve covered very little ground.
For example, AI’s increasing capacity to be better, faster, and cheaper than junior lawyers at entry-level tasks but still nowhere near good enough to substitute for the high-level judgment of senior lawyers raises critical questions of how we turn junior lawyers into senior lawyers if the entry-level work disappears. We did not address such key questions here. Rather, we previously penned a three-part series on new-lawyer training [85], along with contributing to (and completing) Hotshot’s excellent AI courses—part of the new, essential training paradigm includes training on AI itself.
Nor did we get into our bread and butter of actually helping customers navigate the evolving legal innovation ecosystem. The short version of advice we regularly dispense is that near-term efforts should be focused on learning and preparedness, biased towards elegant applications with low switching costs (e.g., Macro) and mature, thick-wrapper products built from the ground up to materially address major pain points (e.g., Dragon). By contrast, we’ve warned customers away from thin-wrapper point solutions, as well as end-to-end platforms that have pretensions of building, and maintaining, the best AI-enabled application for every point in a multi-step process. While platforms have not lost their appeal, the default should be integration-first orientations, such as Agiloft’s “bring your own LLM” capability for CLM [86].
We also left aside the particulars of law department design and spend management. We recently addressed these topics, in nerdy detail, with our Red Team Memo [87]. While everyone has been rightfully distracted getting themselves up to speed on GenAI, the coming contraction (time, budget, headcount) will require increased sophistication. Sophistication entails moving beyond the simple savings math of rate discounts and insourcing, while also taking advantage of all available levers to bend the cost curve: managed services (Factor), marketplaces (Priori), ADR (New Era), invoice review (LegalBillReview.com), experts (Expert Institute), ediscovery (DISCO, Evidence Optix), et cetera.
There is so much to do, on so many different fronts. And there is no time to waste. In light of this overload, wait-and-see on GenAI is ever so tempting, and not entirely illogical—what has been described as “the lazy tyranny of the wait calculation.” [88] It is absolutely true that the longer you wait, the more the application layer will mature, and the more experiments by others will surface what not to do. But, by waiting, you are implicitly placing a bet on how good AI-enabled applications will get (not very), how fast they will get good (not very), and how quickly you will be able to move when the AI is ‘ready’ (quite quickly). These are probably bad bets.
To offer one last analogy, we’ve invented Formula 1 engines (the GenAI models). There’s currently lots of crashing because we’re loading these advanced engines onto golf carts (our existing systems). But fit-for-purpose vehicles (thick-wrapper applications) are being built. In the meantime, it is advisable to get time on the track, if not put yourself in pole position.
In English, even if we hit the pause button on model evolution (not happening), there are massive gains to be made from properly integrating the already powerful models into fit-for-purpose, thick-wrapper applications. While progress can seem slow if you pay close attention to the 24-hour hype machine, when stepping back, the actual rate at which the application layer is maturing is startlingly fast relative to previous technology supercycles. And, again, AI is weird, operating along a jagged, invisible frontier. It requires considerable learning by doing. It demands all kinds of complementary investments in people, process, systems, and data. Unless you are preparing now, you are unlikely to be ready for AI when AI is ready for you. In short, stay ahead or fall behind, probably permanently.
Of course, no enterprise can do it all. Hard choices are inevitable—standing still is a choice with considerable consequences. Strategy is choice. As LexFusion opened one piece on strategy, “Courage is knowing it might hurt and doing it anyway. Stupidity is the same. That’s why life is hard. Strategy is similar… Winning requires knowing you might be wrong and proceeding anyway. Losing is the same. That’s why strategy is hard.” [89]. If you play, you might lose. If you don’t play, you can’t win.
GenAI does not resolve the fundamental tension in strategy [90]. Rather, the far-reaching implications of GenAI make striking the proper balance harder, and more important.
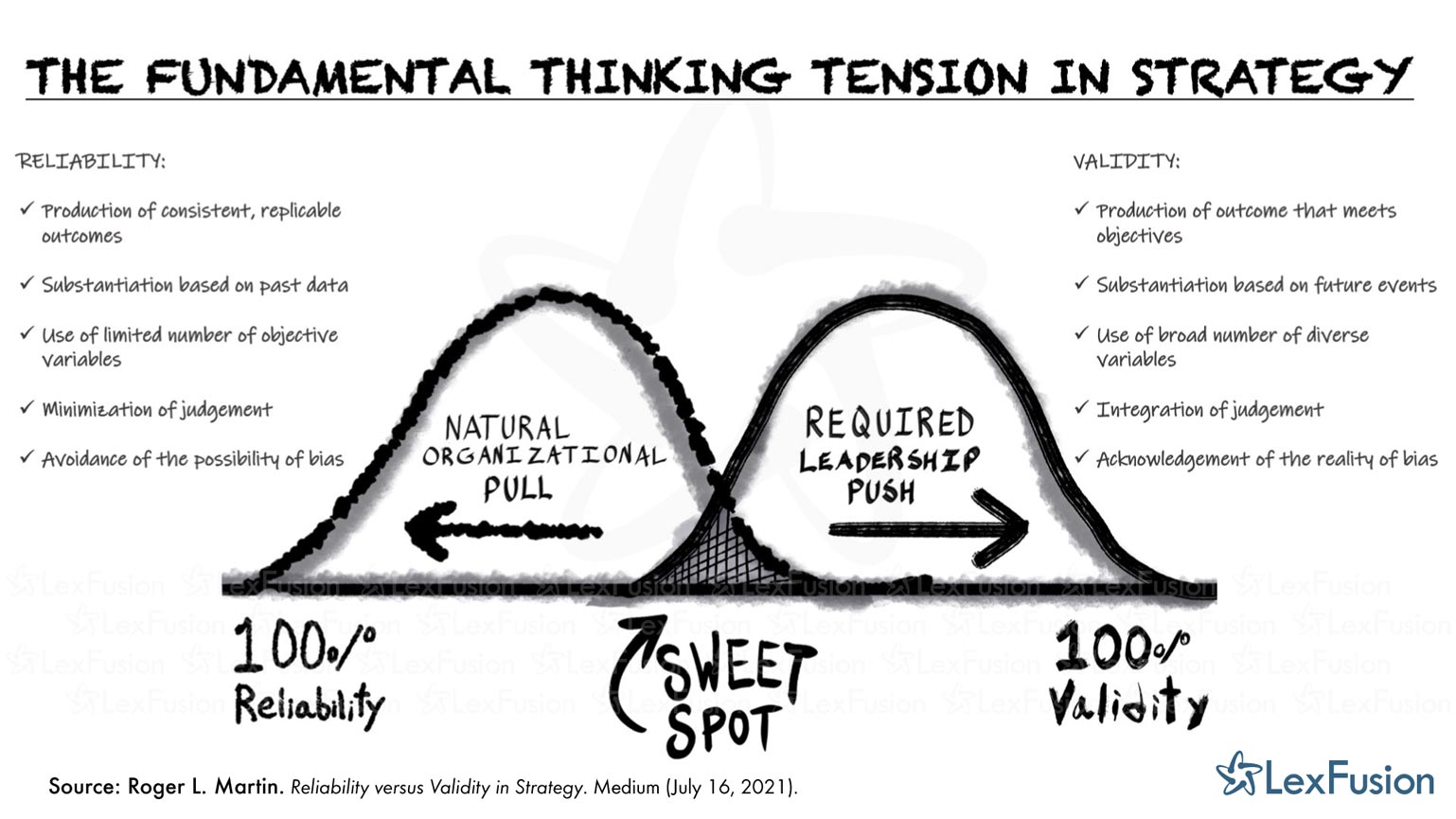
LexFusion is here to help, not to judge. Perfection serves as a useful target but an impossible standard. We’re all in this together. And we can assure you no one else has it all figured out either.
Fortis Fortuna Aduivat
EPILOGUE
Wherever you think AI is headed, you are probably wrong. Us, too. Predictions are hard, especially about the future. The objective of continuous learning is to strive towards being less wrong.
This was a snapshot. But it is meant to be part of a dialogue. Our thinking will evolve. We warmly welcome your feedback, comments, criticisms, and suggestions.
Towards that end, below is Casey’s GPT’s critique of the above. Note the GPT suggests the article be shorter (fair) while also citing the need for more depth, detail, implementation strategies, and case studies (also fair, if incongruent). More ironically, the GPT specifically criticizes the section distinguishing between thin-wrapper and thick-wrapper applications—a section largely written by the same GPT. AI is weird.
PROMPT: Critique the attached. Identify weaknesses, gaps, misperceptions, mischaracterizations, and other shortcomings.
CRITIQUE OF "GENAI IN LEGAL - PROGRESS, PROMISE, PAIN, AND PERIL"
Strengths:
1. Comprehensive Coverage: The article provides an extensive overview of GenAI in legal, covering various perspectives and insights from multiple stakeholders.
2. Practical Insights: It offers practical advice based on real-world use cases, especially from leading law departments and firms.
3. Balanced Viewpoint: The article maintains a balanced perspective, highlighting both the potential and the pitfalls of GenAI in the legal sector.
4. Engaging Format: The use of visuals, quotes, and references to events makes the article engaging and accessible.
Weaknesses:
1. Length and Density: At 30 pages, the article is very long and dense, which might deter busy professionals from reading it in its entirety. A more concise version would be beneficial.
2. Repetition: Some points are repeated throughout the article, which could be streamlined to improve readability and impact.
3. Overemphasis on Hype: While it is important to address the hype around GenAI, the article spends a considerable amount of space reiterating this point, which might have been more effectively condensed.
4. Lack of Clear Structure: The article could benefit from a clearer structure, with distinct sections and subheadings that guide the reader through the content more logically.
Gaps:
1. Insufficient Focus on Specific Use Cases: While the article mentions practical use cases, it could delve deeper into specific examples and detailed outcomes to provide a more concrete understanding.
2. Implementation Strategies: There is limited discussion on actionable implementation strategies for law firms and departments looking to adopt GenAI.
3. Comparison with Other Technologies: A comparative analysis with other emerging technologies in the legal field would provide a broader context and help readers understand where GenAI stands.
Misperceptions and Mischaracterizations:
1. Generalization of Lawyer's Views: The article tends to generalize lawyers' skepticism and resistance to GenAI. It could acknowledge that perspectives vary widely within the legal community.
2. AI Capabilities: At times, the article may overstate or understate AI's capabilities, leading to potential misperceptions about what GenAI can currently achieve versus future potential.
Other Shortcomings:
1. Lack of Technical Detail: For an audience that might include technically savvy readers, the article could provide more detailed technical explanations of how GenAI works and its limitations.
2. Overemphasis on Negative Examples: While it is important to highlight challenges, the article might benefit from balancing these with more success stories and positive outcomes to avoid a predominantly negative tone.
3. Inconsistent Terminology: The use of terms like "thin wrapper" and "thick wrapper" applications could be better explained and consistently applied throughout the article for clarity.
Suggestions for Improvement:
1. Conciseness: Condense the content to focus on key insights and actionable takeaways, reducing redundancy.
2. Clearer Structure: Use clear sections and subheadings to guide readers through the article logically.
3. In-depth Case Studies: Include detailed case studies to provide concrete examples of GenAI implementation and outcomes.
4. Implementation Guide: Provide a step-by-step guide or framework for law firms and departments on how to effectively implement GenAI.
5. Balanced Perspective: Ensure a balanced view by including more success stories and positive impacts of GenAI.
6. Technical Details: Add more technical details for readers interested in the specifics of how GenAI operates and its current limitations.
7. Terminology Clarification: Clarify and consistently use terminology related to AI applications to avoid confusion.
Overall, the article is a valuable resource that could be made more impactful with some targeted revisions to improve clarity, conciseness, and practical utility.
References
- SKILLS 2024, GenAI: A Year in the Trenches
- LexFusion’s Second Annual Legal Market Year in Review
- PSA: ChatGPT is the trailer, not the movie
- And Now, Our Feature Presentation: GPT-4 is Here
- Gen AI’s ‘Collective Brain Trust’ Gathers to Discuss Practical Use Cases and Successes at Invite-Only Event
- Legal Gets Candid About How Gen AI Is Actually Being Used
- How Big Things Get Done
- Hallucinating Law: Legal Mistakes with Large Language Models are Pervasive
- Lawyer cites fake cases invented by ChatGPT, judge is not amused
- Michael Cohen admits fake cases in early release bid came from Google AI program
- Americans’ use of ChatGPT is ticking up, but few trust its election information
- Lawyers’ GenAI Hesitancy: Insights from the 2024 GenAI Professional Services Survey
- Generative AI: now is the time to ‘learn by doing’
- CLE is Broken (as is our approach to learning/innovation)
- Navigating the Jagged Technological Frontier: Field Experimental Evidence of the Effects of AI on Knowledge Worker Productivity and Quality
- Centaurs and Cyborgs on the Jagged Frontier
- Lean back or lean in? Exploring social loafing in human–robot teams
- Two computing paradigms: deterministic and probabilistic (neuromorphic) computing
- A Survey on Hallucination in Large Language Models: Principles, Taxonomy, Challenges, and Open Questions
- Survey reveals AI’s impact on the developer experience
- Reading Between the Lines: Modeling User Behavior and Costs in AI-Assisted Programming
- OpenAI Codex
- Microsoft, GitHub dodge bulk of class action over AI copyright issues
- Adobe Acrobat AI Assistant for enterprise: Our commitment to data governance and security
- What is a context window?
- Lost in the Middle: How Language Models Use Long Contexts
- Chunking Strategies for LLM Applications
- Graph Algorithms and Visualization of Complex Legal Contracts
- The AI Wrapper Dilemma: Choosing Between Thin and Thick Wrappers
- The Easiest Part of LLM Applications is the LLM
- How is GPT-4 at Contract Analysis?
- Latent Expertise: Everyone is in R&D
- What is prompt engineering?
- LLMs can label data as well as humans, but 100x faster
- From data chaos to data products: How enterprises can unlock the power of generative AI
- Rush to Use Generative AI Pushes Companies to Get Data in Order
- Survey: GenAI Is Making Companies More Data Oriented
- The Dirt on Legal Data—and How to Clean It
- Automated Document Review Proves Its Reliability
- Technology-Assisted Review in E-Discovery Can Be More Effective and More Efficient Than Exhaustive Manual Review
- Youngevity Int'l, Corp. v. Smith
- eDiscovery Today 2022 State of the Industry Report
- Survey Report: What factors are driving change in your corporate investigation processes?
- The Sedona Conference Best Practices Commentary on the Use of Search & Information Retrieval Methods in E-Discovery
- Why Facts Don’t Change Our Minds
- Hallucinations Are Legal’s Main Concern With Generative AI, But Maybe Not For Long
- Summarization is (Almost) Dead
- LLM-based summarization: A case study of human, Llama 2 70b and GPT-4 summarization quality
- Better Call GPT, Comparing Large Language Models Against Lawyers
- Why Our Law Firm Bans Generative AI for Research and Writing
- Judging Lawyers: Objectively Evaluating Big Law Litigation Departments
- LinkedIn post re Carlton Fields’ Ban on Generative AI
- U.S. Fifth Circuit Decides Against Its Proposed Rule Amendment on AI Use in Legal Filings
- COMMENTS ON PROPOSED RULE CHANGE TO FIFTH CIRCUIT RULE 32.3 AND FORM 6
- We Need To Talk About GenAI Accuracy
- Deterministic Tests for Complex LLM RAG Applications
- Legal AI Under the Microscope: Stanford HAI’s In-Depth Analysis of Lexis and Westlaw AI Tools
- Evaluating Shepard’s, KeyCite, and Bcite for Case Validation Accuracy
- Everything That Doesn’t Work Yet
- How A Historic Strike Paved the Way for the Automated Elevator and What Those Lessons Could Mean for Self-Driving Cars
- AI that determines risk of death helps save lives in hospital trial
- GenAI and commercial contracting: what’s the point?
- The Bet
- Diffusion of innovations
- Susskind to share views on shifting legal market at ISBA annual meeting
- Wharton Professor Ethan Mollick on the Urgency of Getting in Front of AI
- The State of Generative AI in the Enterprise
- From Potential to Profit with GenAI
- Your Organization Isn’t Designed to Work with GenAI
- Why Adopting GenAI Is So Difficult
- The widespread adoption of AI by companies will take a while
- Artificial intelligence pays off when businesses go all in
- EY CEO Outlook Pulse
- Companies — profitable or not — make 2024 the year of cost cuts
- Legal Departments Want Their Firms Using Gen AI—and Expect Cost Savings
- Jevons paradox
- The Automation Paradox
- Eric Schmidt said ATMs led to more jobs for bank tellers. It’s not that simple.
- We Survived Spreadsheets, and We’ll Survive AI
- How Generative AI Will Transform Knowledge Work
- Sense and Sensibility
- On holding back the strange AI tide
- Legal Tech Trends
- LexFusion Content Library
- LexFusion Content
- Agiloft launches Gen AI Prompt Lab including ‘bring your own LLM’ capability
- LexFusion Red Team Memo
- The Lazy Tyranny of the Wait Calculation
- Strategy and Scale in a Complex World
- Strategy & Leadership #4: Being the Guardian of Validity